Identification of Characteristic lncRNA Molecular Markers in Osteoarthritis by Integrating GEO Database and Machine Learning Strategies and Experimental Validation
-
摘要:目的 利用GEO(Gene Expression Omnibus)数据库联合机器学习筛选骨关节炎(osteoarthritis, OA)特征性的长链非编码RNA(lncRNA)分子标志物。方法 纳入185例OA及76例正常健康人样本,GEO数据库筛选数据集得出差异表达lncRNA,通过随机森林(randomforest, RF)、最小绝对收缩和选择算子(LASSO)逻辑回归、支持向量机递归特征消除(SVM-RFE)3种算法筛选候选的lncRNA模型,绘制受试者操作特征曲线评价模型。收集临床OA患者30例和正常对照15例的外周血,测定免疫炎症指标,RT-PCR定量分析外周血单核细胞lncRNA分子标志物的表达,Pearson分析lncRNA与免疫炎症指标的相关性。结果 LASSO得出14个关键标志物,SVM-RFE算法确定6个基因,RF算法确定24个基因。Venn图筛选得出3种算法的重叠基因,包括HOTAIR、H19、MIR155HG和NKILA。受试者工作特征曲线显示这4个lncRNA的曲线下面积均大于0.7。RT-PCR法发现与正常对照组相比,HOTAIR、H19、MIR155HG在OA患者外周血单核细胞中相对表达量升高,NKILA表达量下降(均P<0.01),结果与生物信息学预测结果相一致。Pearson相关性分析表明选定的lncRNA与临床免疫炎症指标相关。结论 HOTAIR、H19、MIR155HG和NKILA可作为OA临床诊断分子标志物,且与临床免疫炎症指标相关。Abstract:Objective To screen for long non-coding RNA (lncRNA) molecular markers characteristic of osteoarthritis (OA) by utilizing the Gene Expression Omnibus (GEO) database combined with machine learning.Methods The samples of 185 OA patients and 76 healthy individuals as normal controls were included in the study. GEO datasets were screened for differentially expressed lncRNAs. Three algorithms, the least absolute shrinkage and selection operator (LASSO), support vector machine recursive feature elimination (SVM-RFE), and random forest (RF), were used to screen for candidate lncRNA models and receiver operating characteristic (ROC) curves were plotted to evaluate the models. We collected the peripheral blood samples of 30 clinical OA patients and 15 health controls and measured the immunoinflammatory indicators. RT-PCR was performed for quantitative analysis of the expression of lncRNA molecular markers in peripheral blood mononuclear cells (PBMC). Pearson analysis was performed to examine the correlation between lncRNA and indicators for inflammation of the immune system.Results A total of 14 key markers were identified with LASSO, 6 genes were identified with SVM-RFE, and 24 genes were identified with RF. Venn diagram was used to screen for overlapping genes identified with the three algorithms, showing HOTAIR, H19, MIR155HG, and NKILA to be the overlapping genes. The ROC curves showed that these four lncRNAs all had an area under the curve (AUC) greater than 0.7. The RT-PCR findings revealed relatively elevated expression of HOTAIR, H19, and MIR155HG and decreased expression of NKILA in the PBMC of OA patients compared with those of the normal group (P<0.01). The results were consistent with the bioinformatics predictions. Pearson analysis showed that the candidate lncRNAs were correlated with clinical indicators for inflammation.Conclusion HOTAIR, H19, MIR155HG, and NKILA can be used as molecular markers for the clinical diagnosis of OA and are correlate with clinical indicators of inflammation of the immune system.
-
骨关节炎(osteoarthritis, OA)是慢性关节疼痛和残疾的主要原因之一[1]。OA早期诊断困难,目前对OA的治疗限于症状缓解药物和全膝关节置换术,故寻找新的、可行的生物标志物对OA的早期诊断和治疗具有重要意义[2]。
长链非编码RNA(long non-coding, lncRNA)是长度超过200个核苷酸非编码内源RNA,参与OA的发病[3]。lncRNA H19在OA患者外周血[4]中和软骨细胞[5]中高表达,参与炎症反应和软骨细胞代谢;lncRNA HOTAIR在OA患者的外周血单核细胞(peripheral blood mononuclear cell, PBMC)[6]和软骨细胞[7]中均高表达;lncRNA GAS5参与骨疾病的发生发展[8]。这些lncRNA参与OA关节内或外周血免疫炎症细胞浸润过程。免疫浸润的枢纽基因和调控机制是OA研究的热点。文献研究也揭示了外周免疫细胞比值在OA中的预后价值[9]。但鲜有文献研究OA免疫炎症细胞浸润相关的lncRNA分子标志物,及其与OA患者免疫炎症指标的相关性。机器学习则是从大数据中进行算法模型的构建,在疾病亚型识别、生物标志物发现等方面有广泛的应用空间[10]。
本研究从GEO(Gene Expression Omnibus)数据库中获取OA的RNA测序数据,结合机器学习,对差异表达lncRNA进行深度筛选。受试者工作特征(receiver operating characteristic, ROC)曲线评估算法的准确性,以鉴定候选的lncRNA分子诊断标志物。临床试验中加以验证,分析候选lncRNA与OA患者免疫炎症的相关性,以期发现OA中可行的免疫炎症相关生物标志物,为临床OA的早期诊断和治疗提供新的方向。
1. 资料和方法
1.1 数据收集和数据处理
数据集(GEO)数据库(https://www.ncbi.nlm.nih.gov/geo/)的搜索关键词: “Osteoarthritis” “Homo sapiens” “Expression profiling by array”。筛选标准如下:微阵列数据集参考骨关节炎RNA测序数据;包括OA患者样本和健康正常人样本。最终,筛选出5个数据集:GSE43270[11]、GSE51588[12]、GSE117999[13]、GSE169077和GSE48556[14],下载芯片中矩阵及平台文件,共有261个组织样本,包括72个软骨组织(GSE43270、GSE117999和GSE169077),50个胫骨平台样本(GSE51588)和139个外周血单核细胞样本(GSE48556),共包括76个正常健康人样本和185个OA患者样本,具体数据见表1。基于R语言的combat消除多个数据的批次效应[15],RMA(robust multichip average)[16]对数据进行预处理(如表达式计算、归一化、背景校正)。
表 1 基因数据集信息Table 1. Information on the gene datasetsNumber GEO dataset Platform documents NC OA 1 GSE43270 GPL8490 18 23 2 GSE51588 GPL13497 10 40 3 GSE117999 GPL20844 10 10 4 GSE169077 GPL96 5 6 5 GSE48556 GPL6947 33 106 NC: normal control; OA: osteoarthritis. 1.2 差异表达的lncRNA筛选
使用R软件(4.2.0版本)中的Limma包筛选整合的基因表达矩阵中OA的差异表达的lncRNA。以矫正后的adj.P<0.05和|log2FC(fold change)|≥1作为筛选条件,筛选上调和下调的差异基因,基于R中的“RobustRankAggreg, RRA”包对差异表达的lncRNA进行进一步识别,得到稳定的lncRNA分子标志物。这种RRA方法可以最小化多个数据集的偏差。
1.3 候选的lncRNA分子标志物的筛选与验证
3种算法被用于筛选候选的lncRNA,包括随机森林(randomforest, RF)[17]、最小绝对收缩和选择算子(LASSO)逻辑回归[18]、支持向量机递归特征消除(SVM-RFE)[19]。RF算法运用R包“randomForest”(包含mtry和trees参数)构建RF分类识别模型,其中mtry参数的取值方法为输入变量数量开平方根,trees为RF中包含的决策树数目(默认为500)。使用R包“glmnet”进行LASSO逻辑回归调查。该法有赖于交叉验证(cross validation)方法筛选参数,方法如下:用训练集(正常健康人数据)算参数及系数,用验证集(OA患者数据)来算对应的残差平方和,然后根据10次交叉验证结果的残差平方和的平均值选取LASSO中参数,binomial回归算法得最优模型,最小lambda被认为是最优的。SVM-RFE算法是采用5倍交叉验证的方法,对插入符号包中具有RFE功能的特征基因进行筛选。利用训练数据集训练SVM,得到相应的参数;选择公式(1)的排序准则计算所有特征在该准则下的排序准则分数;在验证集中剔除对应于最小得分的特征。执行上述过程进行5次交叉验证。这种特征选择的过程实际上是一个序列后向选择(sequence backward selection, SBS)的过程。排序准则公式如(1),其中
$ {W^2} $ 和${W^{ - (P)2}}$ 分别表示完整SVM的权重和假设剔除第P个特征后SVM的权重,$ {W^2} $ 的表示形式如(2),i、j表示循环变量;y表示类别标记;N表示样本数目;$ K({\chi }_{{i},}{\chi }_{j}) $ 表示核函数;$ \alpha _i^* $ 和$ \alpha _j^* $ 由解算SVM对偶优化问题得到。绘制SVM-error和SVM-Accuracy图形。上述过程都是基于R包构建。然后,维恩图(venn diagram, Venn)绘制上述3种分类模型中的重叠基因。为了深入检测重叠基因的功性,在ROC曲线调查的基础上进行评估,并计算曲线下面积(area under the curve, AUC),评估算法的预测效果。双侧P<0.05为差异有统计学意义。$$ {R_c} = \left| {{W^2}} \right. - {W^{ - (P)}}{^2}\left. \right| $$ (1) $$ {W^2} = \sum\limits_{i,j - 1}^N {\alpha _i^*} \alpha _j^*{y_i}{y_j}K({\chi _i},{\chi _j}) $$ (2) 1.4 临床标本收集
本研究选取安徽中医药大学第一附属医院风湿免疫科于2022年11月–2023年2月确诊的30例未接受药物治疗的OA患者。诊断标准参考2018年中华医学会风湿病学分会修订的《骨关节炎诊断及治疗指南》[20]。X线检查根据Kellgren-Lawrence标准[21]符合1、2、3级的患者。其中11名男性,19名女性,平均年龄为(56.63±12.06)岁。此外,还招募了同期体检中心健康个体作为对照组,排除标准:①合并骨关节炎、类风湿关节炎等风湿病的患者;②合并循环系统、呼吸系统、造血系统等疾病的患者;③孕妇或哺乳期女性的患者;④精神病患者。对年龄、性别进行一对一倾向性匹配,纳入15例作为对照组,其中8例男性,12例女性,平均年龄为(53.60±7.72)岁。两组一般资料差异无统计学意义。安徽中医药大学附属第一医院伦理委员会对这项工作进行了评审和批准(伦理号:No. 2022MCZQ01)。
1.5 免疫炎症指标测定
采用全自动生化分析仪(日立HITACHI7600)检测全血生化指标,包括免疫球蛋白A(immunoglobulin A, IgA)、C反应蛋白(C-reactive protein, CRP)、白细胞介素6(interleukin 6, IL-6)、补体4(complement 4, C4)、补体3(complement 3, C3)、免疫球蛋白M(immunoglobulin M, IgM)、红细胞沉降率(erythrocyte sedimentation rate, ESR)、免疫球蛋白E(immunoglobulin E, IgE)、免疫球蛋白G(immunoglobulin G, IgG)。
1.6 RT-PCR定量分析
采用密度梯度离心法分离两组样本PBMC。收集细胞沉淀,用Trizol法提取RNA。RNA的数量使用Nano Drop分光光度计(NanoDrop Technologies, Wilmington, NC, USA)测量。RT试剂盒中使用gDNA Eraser(TaKaRa, Shiga, Japan)生成cDNA。表2列出了qRT-PCR中使用的引物。内参基因为GAPDH。相对表达值均计算为2−ΔΔCt。
表 2 特异基因引物序列Table 2. Specific gene primer sequencesGene Forward primer (5′→3′) Reverse primer (5′→3′) GAPDH TTCCACCCATGGCAAATTCC ATCTCGCTCCTGGAAGATGG MIR155HG GAGTGCTGAAGGCTTGCTGT TTGAACATCCCAGTGACCAG HOTAIR GGAAAGATCCAAATGGGACC CTAGGAATCAGCACGAAGCA H19 TGATGACGGGTGGAGGGGCT TGATGTCGCCCTGTCTGCAC NKILA CTGTCGGGGACTGGTGTATT AATACACCAGTCCCCGACAG GAPDH: glyceraldehyde-3-phosphate dehydrogenase; MIR155HG: MIR155 host gene; HOTAIR: HOX transcript antisense RNA; H19: H19 imprinted maternally expressed transcript; NKILA: NF-kappa B interacting lncRNA. 1.7 统计学方法
计量资料采用
$ \bar x \pm s $ 表示,所有样本均用Kolmogorov-Smirnov检验正态性,若符合正态性和方差齐的数据,组间比较采用两独立样本t检验,不符合则选取非参数检验。相关性分析采用Spearman或Pearson分析。一对一倾向性匹配法运用SPSS中倾向匹配得分工具实现。 P<0.05为差异有统计学意义。2. 结果
2.1 差异表达的lncRNA识别
对5组数据集进行了标准化处理,从图1A看出不同的批次之间各自成团,批次之间有比较明显的差别,存在一定的批次效应。图1B不同批次的样品重叠在一起,表明批次效应校正成功。使用OriginLab构建整合基因集中差异表达lncRNA的火山图,见图2。进一步通过R语言RRA法筛选出105个差异lncRNA,包括30个上调和75个下调的lncRNA。将所得差异表达lncRNA对应统计量进行汇总,按校正后的P值即adj.P.Val从小到大进行排序,一般认为adj.P.Val值越小代表差异表达越显著,依据adj.P.Val值选择差异表达最显著的前10个lncRNA,见表3。
表 3 差异表达最显著的前10个lncRNATable 3. Top 10 lncRNAs showing the most significant difference in their expressionIndex GEO data set Gene log2FC P.Value adj.P.Val 1 GSE51588 MIR155HG 9.581 4.44E-03 9.05E-02 GSE117999 GSE48556 2 GSE51588 HOTAIR 2.321 6.44E-06 9.90E-04 GSE117999 GSE48556 3 GSE48556 NKILA −3.686 1.46E-05 1.26E-02 GSE169077 4 GSE43270 H19 2.216 3.05E-05 1.34E-02 GSE51588 GSE117999 GSE48556 5 GSE43270 MEG3 −3.033 3.01E-05 1.34E-02 GSE51588 GSE117999 GSE48556 6 GSE48556 LINC00973 2.146 3.76E-05 1.36E-02 7 GSE51588 C15orf54 −2.013 8.44E-05 2.01E-02 GSE117999 GSE48556 8 GSE117999 MEG9 2.252 1.33E-04 2.43E-02 9 GSE43270 PART1 2.191 1.73E-03 6.23E-02 GSE51588 GSE117999 GSE48556 10 GSE51588 C3orf79 2.179 2.10E-03 6.67E-02 GSE117999 2.2 候选的lncRNA分子标志物的筛选与验证
运用3种算法对候选的105个差异lncRNA进行识别,其中LASSO算法得出14个关键的生物标志物(图3)。SVM-RFE算法确定了6个基因作为必要的生物标志物(图4),图4A是5倍交叉验证后曲线变化的错误率,图中6-0.173表示筛选出的6个特征性基因的错误率是0.173,越接近0,表明错误率越低;图4B是5倍交叉验证后曲线变化的准确率,图中6-0.827表示筛选出6个特征性基因的准确率是0.827,越接近1,表明准确率越高。除此之外,RF算法认为24个基因是重要的生物标志物(图5A,5B)。Venn图绘制3种算法的重叠基因(图6A),最终得到4个lncRNA,其中HOTAIR、H19、MIR155HG上调(log2FC≥1),NKILA下调( log2FC<−1)。ROC曲线显示,它们可能是有价值的生物标志物,AUC分别为0.9415〔95%置信区间(confidence interval, CI):0.9010~0.9820〕(HOTAIR)、0.8016(95%CI:0.7244~0.8788)(H19)、0.9751(95%CI:0.9475~0.9991)(MIR155HG)、0.7957(95%CI:0.7165~0.8748)(NKILA)(图6B)。
图 3 LASSO算法筛选14个lncRNAFigure 3. LASSO algorithm was used to screen out 14 lncRNAsA, Each curve in the figure represents the change trajectory of each independent variable coefficient, the vertical coordinate is the value of the coefficient, the lower horizontal coordinate is log (λ), and the upper horizontal coordinate is the number of non-zero coefficients in the model at this time. B, The vertical coordinate is Binomial Deviance (dichotomous anomaly), which can be interpreted as the magnitude of the error of the model. There are two dashed lines of values in the figure, the left is the line with the lowest error and the right is the line with fewer features.图 4 SVM-RFE算法筛选出6个关键lncRNAFigure 4. Support vector machine recursive feature elimination (SVM-RFE) algorithm was used to screen out 6 key lncRNAsGraph A is SVM error and graph B is SVM accuracy. 5×CV represents 5-fold cross-validation. The number 6-0.173 in Fig 4A indicates that the error rate for the six trait genes screened out was 0.173. The number 6-0.827 in Fig 4B indicates that the accuracy rate of the six trait genes screened out was 0.827.图 5 RF算法筛选24个特征lncRNAFigure 5. Random forest (RF) algorithm was used to screen out 24 feature lncRNAsA, The dynamics of the random forest prediction error versus the number of random trees, with the vertical axis of error representing the error; the horizontal axis of trees representing the tree number. The black, red, and green lines show how the false positive rate varies with the number of decision trees for all samples, samples from osteoarthritis patients, and samples from normal healthy people in the five datasets, respectively. B, The 24 genes sorted by importance.2.3 临床患者免疫炎症指标变化
与正常对照组相比,OA患者中炎症指标(ESR、CRP、IL-6)、免疫球蛋白(IgA、IgE)、补体C4升高,差异有统计学意义(P<0.05)。见表4。
表 4 两组免疫炎症指标的变化Table 4. Changes in immunoinflammatory indicators in the two groupsIndicator NC group (n=15) OA group (n=30) P ESR/(mm/1 h) 3.45±1.34 15.6±7.34 <0.001 CRP/(mg/L) 0.73±0.56 8.3±4.24 <0.001 IgA/(g/L) 1.68±0.22 3.73±1.25 <0.001 IgM/(g/L) 1.04±0.12 1.25±0.65 0.654 IgG/(g/L) 11.47±3.45 13.79±6.44 0.545 IgE/(IU/mL) 19.49±9.45 70.56±15.56 0.013 C3/(g/L) 0.63±0.12 0.84±0.32 0.576 C4/(g/L) 0.11±0.11 0.76±0.89 0.021 IL-6/(pg/mL) 2.38±1.45 13.09±3.56 0.011 ESR: erythrocyte sedimentation rate; CRP: C-reactive protein; IgA: immunoglobulin A; IgM: immunoglobulin M; IgG: immunoglobulin G; IgE: immunoglobulin E; C3: complement 3; C4: complement 4; IL-6: interleukin 6. The other abbreviations are explained in the notes to Table 1. 2.4 RT-PCR法检测lncRNA分子标志物的表达和相关性分析
RT-PCR结果显示,与正常人相比,OA患者PBMC中HOTAIR、H19、MIR155HG相对表达量升高(P<0.01),NKILA相对表达量下降(P<0.01),与生物信息学分析结果一致(图7)。Pearson相关性分析表明,H19与IgA(r=0.439,P=0.018)呈正相关,MIR155HG与CRP(r=0.785,P<0.001)、IgM(r=0.454,P=0.008)、IL-6(r=0.610,P<0.001)呈正相关,NKILA与ESR(r=−0.425,P=0.021)呈负相关,与IL-6(r=0.650,P<0.001)呈正相关,HOTAIR与CRP(r=0.589,P=0.001)、IL-6(r=0.492,P=0.006)和IgE(r=0.445,P=0.014)呈正相关。表明筛选出的4个特征性lncRNA与免疫炎症指标存在相关性(表5)。
表 5 lncRNA分子标志物与免疫炎症指标的Pearson分析Table 5. Pearson analysis of lncRNA molecular markers and immunoinflammatory indicatorsIndicator H19 MIR155HG NKILA HOTAIR r P r P r P r P ESR/(mm/1 h) 0.044 0.816 0.355 0.052 −0.425 0.021 0.345 0.054 CRP/(mg/L) 0.014 0.941 0.785 <0.001 −0.308 0.064 0.589 0.001 IgA/(g/L) 0.439 0.018 0.220 0.243 −0.312 0.056 0.212 0.260 IgM/(g/L) 0.298 0.110 0.454 0.008 −0.063 0.742 0.040 0.834 IgG/(g/L) 0.090 0.637 0.119 0.531 −0.122 0.522 0.095 0.618 IgE/(IU/mL) 0.358 0.051 0.008 0.968 −0.183 0.333 0.445 0.014 C3/(g/L) 0.035 0.856 0.212 0.260 −0.194 0.304 0.214 0.247 C4/(g/L) 0.028 0.883 0.010 0.960 −0.007 0.972 0.221 0.214 IL-6/(pg/mL) 0.061 0.749 0.610 <0.001 0.650 <0.001 0.492 0.006 ESR, CRP, IgA, IgM, IgG, IgE, C3, C4 and IL-6 denote the same as those in Table 4. H19, MIR155HG, NKILA and HOTAIR denote the same as those in Table 2. 3. 讨论
OA的发生发展涉及复杂的生物学反应[22]。由于缺乏早期检测和评估治疗结果的有效方法,目前,生物标志物的发现成为辅助疾病监测的前瞻性方法。本研究基于GEO测序数据集,结合机器学习策略,寻找OA新的免疫炎症相关的分子标志物,并在OA患者外周血标本中进行验证,为OA的早期诊断和治疗提供新的思路和研究方向。
在本研究中,通过筛选5个OA软骨细胞全基因组基因表达谱,整合差异表达的lncRNA,确定了105个差异lncRNA,包括30个上调和75个下调的lncRNA。根据adj.P.Val值从小到大排序,表3列出前10的lncRNA,包括下调的NKILA、MEG3和C15orf54(log2FC<-1),上调的MIR155HG、HOTAIR、H19、LINC00973、MEG9、PART1和C3orf79(log2FC≥1)。 还有一些lncRNA值得关注,如上调的XIST[23]、TUG1[24]、DANCR[25]、MIAT[26],下调的MEG3[27]、THRIL[28]、GAS5[8]、ATB[29]。这些lncRNA可能与OA的发病机制有关。
通过集成3种不同的算法,得到3种算法的重叠基因,分别是HOTAIR、H19、MIR155HG和NKILA。ROC曲线结果也表明,4个生物标志物AUC均大于0.7,表明预测结果具有较强的准确性。HOTAIR在OA组织中高表达,通过抑制软骨细胞增殖,促进细胞凋亡和细胞外基质降解导致软骨细胞的功能障碍[30]。lncRNA NKILA通过与核因子κB/核因子κB的抑制蛋白(nuclear factor kappa-B/inhibitor of NF-κB, NF-κB/IκB)复合物结合来覆盖IκB的磷酸化位点,从而抑制NF-κB的过度活化[31]。lncRNA MIR155HG也称为B细胞整合簇。MIR155HG的表达与免疫细胞、分子和免疫检查点分子的浸润水平显著相关[32]。MIR155HG参与破骨细胞的调节。在骨质疏松小鼠中,MIR155HG通过AMP依赖的蛋白激酶(AMP-activated protein kinase, AMPK)途径靶向瘦素受体基因来抑制破骨细胞活化[33]。MIR155HG上调可能是OA炎症过程的重要因素。lncRNA H19在OA中高度表达,可能通过白介素(Interleukin,IL)-38和IL-36之间的相互作用促进OA中的炎症反应[5]。综上所述,基于3种算法的机器学习组合模型和文献调研,均表明HOTAIR、H19、MIR155HG和NKILA与OA中免疫炎症存在相关性,可作为特征性诊断的标志物,但其诊断意义还需要大量的实验来验证。
临床样本验证结果显示,与正常健康组相比,HOTAIR、H19、MIR155HG相对表达量升高,NKILA相对表达量下降,结果与生物信息学预测结果相一致,表明本研究整合预测策略的可行性。相关性分析显示4个特征性lncRNA与免疫炎症指标的相关性。IL-6在软骨病理的发展中起着关键作用,OA患者血清或滑液中IL-6水平的增加与疾病的严重程度相关;然而,IL-6也增加了抗分解代谢因子的表达,具有保护作用;表明IL-6在OA中起双重作用,可能是由IL-6经典与反式信号传导的不同效应引起的[34]。IgE介导的肥大细胞通过高亲和力IgE受体(FcεRI),导致促炎细胞和降解介质(包括类胰蛋白酶)的释放,导致组织损伤、炎症和肥大细胞活化,导致OA的发展和进展[35]。CRP和ESR可作为炎症反应的非特异性指标[36]。研究发现CRP是影响OA诊断和严重程度分级的独立因素[37]。免疫球蛋白亚型在OA患者血清中表达升高,参与了OA发病过程[38]。例如,IgA[39]和 IgM[40]作为机体内高亲和力抗体,参与免疫应答反应。相关性分析也表明,MIR155HG、NKILA、HOTAIR与炎症指标(CRP、ESR和IL-6)、免疫指标(IgE、IgA和IgM)存在相关性,表明特征性的lncRNA参与了OA的免疫炎症反应,在OA临床患者应用中有重要意义。
本研究预测的结果来源于GEO数据库多个实验测序数据的组合,多个算法整合机器学习策略来识别特征性基因,信息利用度方面较单个数据集和小样本的实验验证更全面和完善。然而,本研究存在一定的局限性:样本量相对较小,软骨标本难获取,因此选择外周血标本进行验证。此外,OA患者之间的个体差异,包括社会经济地位、疾病严重程度和疾病持续时间,可能会影响结果的准确性。因此,进一步大样本的体内外实验验证特征性的lncRNA在OA免疫炎症中的确切机制将是我们下一步研究的目标。
* * *
作者贡献声明 刘健负责论文构思,忻凌负责正式分析,周巧和齐亚军负责调查研究,周巧、方妍妍和胡月迪负责可视化,周巧负责初稿写作和审读与编辑写作。所有作者已经同意将文章提交给本刊,且对将要发表的版本进行最终定稿,并同意对工作的所有方面负责。
利益冲突 所有作者均声明不存在利益冲突
-
图 3 LASSO算法筛选14个lncRNA
Figure 3. LASSO algorithm was used to screen out 14 lncRNAs
A, Each curve in the figure represents the change trajectory of each independent variable coefficient, the vertical coordinate is the value of the coefficient, the lower horizontal coordinate is log (λ), and the upper horizontal coordinate is the number of non-zero coefficients in the model at this time. B, The vertical coordinate is Binomial Deviance (dichotomous anomaly), which can be interpreted as the magnitude of the error of the model. There are two dashed lines of values in the figure, the left is the line with the lowest error and the right is the line with fewer features.
图 4 SVM-RFE算法筛选出6个关键lncRNA
Figure 4. Support vector machine recursive feature elimination (SVM-RFE) algorithm was used to screen out 6 key lncRNAs
Graph A is SVM error and graph B is SVM accuracy. 5×CV represents 5-fold cross-validation. The number 6-0.173 in Fig 4A indicates that the error rate for the six trait genes screened out was 0.173. The number 6-0.827 in Fig 4B indicates that the accuracy rate of the six trait genes screened out was 0.827.
图 5 RF算法筛选24个特征lncRNA
Figure 5. Random forest (RF) algorithm was used to screen out 24 feature lncRNAs
A, The dynamics of the random forest prediction error versus the number of random trees, with the vertical axis of error representing the error; the horizontal axis of trees representing the tree number. The black, red, and green lines show how the false positive rate varies with the number of decision trees for all samples, samples from osteoarthritis patients, and samples from normal healthy people in the five datasets, respectively. B, The 24 genes sorted by importance.
表 1 基因数据集信息
Table 1 Information on the gene datasets
Number GEO dataset Platform documents NC OA 1 GSE43270 GPL8490 18 23 2 GSE51588 GPL13497 10 40 3 GSE117999 GPL20844 10 10 4 GSE169077 GPL96 5 6 5 GSE48556 GPL6947 33 106 NC: normal control; OA: osteoarthritis. 表 2 特异基因引物序列
Table 2 Specific gene primer sequences
Gene Forward primer (5′→3′) Reverse primer (5′→3′) GAPDH TTCCACCCATGGCAAATTCC ATCTCGCTCCTGGAAGATGG MIR155HG GAGTGCTGAAGGCTTGCTGT TTGAACATCCCAGTGACCAG HOTAIR GGAAAGATCCAAATGGGACC CTAGGAATCAGCACGAAGCA H19 TGATGACGGGTGGAGGGGCT TGATGTCGCCCTGTCTGCAC NKILA CTGTCGGGGACTGGTGTATT AATACACCAGTCCCCGACAG GAPDH: glyceraldehyde-3-phosphate dehydrogenase; MIR155HG: MIR155 host gene; HOTAIR: HOX transcript antisense RNA; H19: H19 imprinted maternally expressed transcript; NKILA: NF-kappa B interacting lncRNA. 表 3 差异表达最显著的前10个lncRNA
Table 3 Top 10 lncRNAs showing the most significant difference in their expression
Index GEO data set Gene log2FC P.Value adj.P.Val 1 GSE51588 MIR155HG 9.581 4.44E-03 9.05E-02 GSE117999 GSE48556 2 GSE51588 HOTAIR 2.321 6.44E-06 9.90E-04 GSE117999 GSE48556 3 GSE48556 NKILA −3.686 1.46E-05 1.26E-02 GSE169077 4 GSE43270 H19 2.216 3.05E-05 1.34E-02 GSE51588 GSE117999 GSE48556 5 GSE43270 MEG3 −3.033 3.01E-05 1.34E-02 GSE51588 GSE117999 GSE48556 6 GSE48556 LINC00973 2.146 3.76E-05 1.36E-02 7 GSE51588 C15orf54 −2.013 8.44E-05 2.01E-02 GSE117999 GSE48556 8 GSE117999 MEG9 2.252 1.33E-04 2.43E-02 9 GSE43270 PART1 2.191 1.73E-03 6.23E-02 GSE51588 GSE117999 GSE48556 10 GSE51588 C3orf79 2.179 2.10E-03 6.67E-02 GSE117999 表 4 两组免疫炎症指标的变化
Table 4 Changes in immunoinflammatory indicators in the two groups
Indicator NC group (n=15) OA group (n=30) P ESR/(mm/1 h) 3.45±1.34 15.6±7.34 <0.001 CRP/(mg/L) 0.73±0.56 8.3±4.24 <0.001 IgA/(g/L) 1.68±0.22 3.73±1.25 <0.001 IgM/(g/L) 1.04±0.12 1.25±0.65 0.654 IgG/(g/L) 11.47±3.45 13.79±6.44 0.545 IgE/(IU/mL) 19.49±9.45 70.56±15.56 0.013 C3/(g/L) 0.63±0.12 0.84±0.32 0.576 C4/(g/L) 0.11±0.11 0.76±0.89 0.021 IL-6/(pg/mL) 2.38±1.45 13.09±3.56 0.011 ESR: erythrocyte sedimentation rate; CRP: C-reactive protein; IgA: immunoglobulin A; IgM: immunoglobulin M; IgG: immunoglobulin G; IgE: immunoglobulin E; C3: complement 3; C4: complement 4; IL-6: interleukin 6. The other abbreviations are explained in the notes to Table 1. 表 5 lncRNA分子标志物与免疫炎症指标的Pearson分析
Table 5 Pearson analysis of lncRNA molecular markers and immunoinflammatory indicators
Indicator H19 MIR155HG NKILA HOTAIR r P r P r P r P ESR/(mm/1 h) 0.044 0.816 0.355 0.052 −0.425 0.021 0.345 0.054 CRP/(mg/L) 0.014 0.941 0.785 <0.001 −0.308 0.064 0.589 0.001 IgA/(g/L) 0.439 0.018 0.220 0.243 −0.312 0.056 0.212 0.260 IgM/(g/L) 0.298 0.110 0.454 0.008 −0.063 0.742 0.040 0.834 IgG/(g/L) 0.090 0.637 0.119 0.531 −0.122 0.522 0.095 0.618 IgE/(IU/mL) 0.358 0.051 0.008 0.968 −0.183 0.333 0.445 0.014 C3/(g/L) 0.035 0.856 0.212 0.260 −0.194 0.304 0.214 0.247 C4/(g/L) 0.028 0.883 0.010 0.960 −0.007 0.972 0.221 0.214 IL-6/(pg/mL) 0.061 0.749 0.610 <0.001 0.650 <0.001 0.492 0.006 ESR, CRP, IgA, IgM, IgG, IgE, C3, C4 and IL-6 denote the same as those in Table 4. H19, MIR155HG, NKILA and HOTAIR denote the same as those in Table 2. -
[1] ZHOU Q, LIU J, XIN L, et al. Exploratory compatibility regularity of Traditional Chinese Medicine on osteoarthritis treatment: a data mining and random walk-based identification. Evid Based Complement Alternat Med,2021,2021: 2361512. DOI: 10.1155/2021/2361512
[2] LI J, YANG X, CHU Q, et al. Multi-omics molecular biomarkers and database of osteoarthritis. Database (Oxford),2022,2022: baac052. DOI: 10.1093/database/baac052
[3] 仇学梅, 李鑫, 刘锐. 非编码RNA与先天免疫信号调控. 四川大学学报(医学版),2022,53(1): 20–27. DOI: 10.12182/20220160202 [4] ZHOU L, WAN Y, CHENG Q, et al. The expression and diagnostic value of lncRNA H19 in the blood of patients with osteoarthritis. Iran J Public Health,2020,49(8): 1494–1501. DOI: 10.18502/ijph.v49i8.3893
[5] ZHOU Y, LI J, XU F, et al. Long noncoding RNA H19 alleviates inflammation in osteoarthritis through interactions between TP53, IL-38, and IL-36 receptor. Bone Joint Res,2022,11(8): 594–607. DOI: 10.1302/2046-3758.118.BJR-2021-0188.R1
[6] CHEN X, LIU J, SUN Y, et al. Correlation analysis of differentially expressed long non-coding RNA HOTAIR with PTEN/PI3K/AKT pathway and inflammation in patients with osteoarthritis and the effect of baicalin intervention. J Orthop Surg Res,2023,18(1): 34. DOI: 10.1186/s13018-023-03505-1
[7] HU J, WANG Z, SHAN Y, et al. Long non-coding RNA HOTAIR promotes osteoarthritis progression via miR-17-5p/FUT2/β-catenin axis. Cell Death Dis,2018,9(7): 711. DOI: 10.1038/s41419-018-0746-z
[8] ZHOU Z, CHEN J, HUANG Y, et al. Long noncoding RNA GAS5: a new factor involved in bone diseases. Front Cell Dev Biol,2022,26(9): 807419. DOI: 10.3389/fcell.2021.807419
[9] CULEMANN S, GRUNEBOOM A, KRONKE G. Origin and function of synovial macrophage subsets during inflammatory joint disease. Adv Immunol,2019,143: 75–98. DOI: 10.1016/bs.ai.2019.08.006
[10] ZHANG Q, SUN C, LIU X, et al. Mechanism of immune infiltration in synovial tissue of osteoarthritis: a gene expression-based study. J Orthop Surg Res,2023,18(1): 58. DOI: 10.1186/s13018-023-03541-x
[11] FERNANDEZ-TAJES J, SOTO-HERMIDA A, VAZQUEZ-MOSQUERA M E, et al. Genome-wide DNA methylation analysis of articular chondrocytes reveals a cluster of osteoarthritic patients. Ann Rheum Dis,2014,73(4): 668–677. DOI: 10.1136/annrheumdis-2012-202783
[12] CHOU C H, WU C C, SONG I W, et al. Genome-wide expression profiles of subchondral bone in osteoarthritis. Arthritis Res Ther,2013,15(6): R190. DOI: 10.1186/ar4380
[13] BROPHY R H, ZHANG B, CAI L, et al. Transcriptome comparison of meniscus from patients with and without osteoarthritis. Osteoarthritis Cartilage,2018,26(3): 422–432. DOI: 10.1016/j.joca.2017.12.004
[14] RAMOS Y F, BOS S D, LAKENBERG N, et al. Genes expressed in blood link osteoarthritis with apoptotic pathways. Ann Rheum Dis,2014,73(10): 1844–1853. DOI: 10.1136/annrheumdis-2013-203405
[15] RADUA J, VIETA E, SHINOHARA R, et al. Increased power by harmonizing structural MRI site differences with the ComBat batch adjustment method in ENIGMA. Neuroimage,2020,218: 116956. DOI: 10.1016/j.neuroimage.2020.116956
[16] PEIGNIER S, CALEVRO F. Gene self-expressive networks as a generalization-aware tool to model gene regulatory networks. Biomolecules,2023,13(3): 526. DOI: 10.3390/biom13030526
[17] SPEISER J L. A random forest method with feature selection for developing medical prediction models with clustered and longitudinal data. J Biomed Inform,2021,117: 103763. DOI: 10.1016/j.jbi.2021.103763
[18] LEVY J J, O'MALLEY A J. Don't dismiss logistic regression: the case for sensible extraction of interactions in the era of machine learning. BMC Med Res Methodol,2020,20(1): 171. DOI: 10.1186/s12874-020-01046-3
[19] LIN X, LI C, ZHANG Y, et al. Selecting feature subsets based on SVM-RFE and the overlapping ratio with applications in bioinformatics. Molecules,2017,23(1): 52. DOI: 10.3390/molecules23010052
[20] 高宏伟, 于东旭, 韩继成, 等. 基于循证医学指南的膝关节骨关节炎非手术诊疗方案思考. 长春中医药大学学报,2022,38(9): 952–955. DOI: 10.13463/j.cnki.cczyy.2022.09.003 [21] OLSSON S, AKBARIAN E, LIND A, et al. Automating classification of osteoarthritis according to Kellgren-Lawrence in the knee using deep learning in an unfiltered adult population. BMC Musculoskelet Disord,2021,22(1): 844. DOI: 10.1186/s12891-021-04722-7
[22] HAUBRUCK P, PINTO M M, MORADI B, et al. Monocytes, macrophages, and their potential niches in synovial joints-therapeutic targets in post-traumatic osteoarthritis? Front Immunol,2021,12: 763702. DOI: 10.3389/fimmu.2021.763702
[23] CHEN H, YANG S, SHAO R. Long non-coding XIST raises methylation of TIMP-3 promoter to regulate collagen degradation in osteoarthritic chondrocytes after tibial plateau fracture. Arthritis Res Ther,2019,21(1): 271. DOI: 10.1186/s13075-019-2033-5
[24] HAN H, LIN L. Long noncoding RNA TUG1 regulates degradation of chondrocyte extracellular matrix via miR-320c/MMP-13 axis in osteoarthritis. Open Life Sci,2021,16(1): 384–394. DOI: 10.1515/biol-2021-0037
[25] ZHANG L, ZHANG P, SUN Y, et al. Long non-coding RNA DANCR regulates proliferation and apoptosis of chondrocytes in osteoarthritis via miR-216a-5p-JAK2-STAT3 axis. Biosci Rep,2018,138(6): BSR20181228. DOI: 10.1042/BSR20181228
[26] LI R, SHI T T, WANG Q, et al. Elevated lncRNA MIAT in peripheral blood mononuclear cells contributes to post-menopausal osteoporosis. Aging (Albany NY),2022,14(7): 3143–3154. DOI: 10.18632/aging.204001
[27] WANG A, HU N, ZHANG Y, et al. MEG3 promotes proliferation and inhibits apoptosis in osteoarthritis chondrocytes by miR-361-5p/FOXO1 axis. BMC Med Genomics,2019,12(1): 201. DOI: 10.1186/s12920-019-0649-6
[28] ZOU Y, SHEN C, SHEN T, et al. lncRNA THRIL is involved in the proliferation, migration, and invasion of rheumatoid fibroblast-like synoviocytes. Ann Transl Med,2021,9(17): 1368. DOI: 10.21037/atm-21-1362
[29] DANG X, WU D. The diagnostic value and pathogenetic role of lncRNA-ATB in patients with osteoarthritis. Cell Mol Biol Lett,2018,27(23): 55. DOI: 10.1186/s11658-018-0118-9
[30] LU J, WU Z, XIONG Y. Knockdown of long noncoding RNA HOTAIR inhibits osteoarthritis chondrocyte injury by miR-107/CXCL12 axis. J Orthop Surg Res,2021,16(1): 410. DOI: 10.1186/s13018-021-02547-7
[31] HU D, ZHONG T, DAI Q. Long non-coding RNA NKILA reduces oral squamous cell carcinoma development through the NF-KappaB signaling pathway. Technol Cancer Res Treat,2020,19: 1533033820960747. DOI: 10.1177/1533033820960747
[32] PENG L, CHEN Z, CHEN Y, et al. MIR155HG is a prognostic biomarker and associated with immune infiltration and immune checkpoint molecules expression in multiple cancers. Cancer Med,2019,8(17): 7161–7173. DOI: 10.1002/cam4.2583
[33] MAO Z, ZHU Y, HAO W, et al. MicroRNA-155 inhibition up-regulates LEPR to inhibit osteoclast activation and bone resorption via activation of AMPK in alendronate-treated osteoporotic mice. IUBMB Life,2019,71(12): 1916–1928. DOI: 10.1002/iub.2131
[34] WIEGERTJES R, Van De LOO F A J, BLANEY DAVIDSON E N. A roadmap to target interleukin-6 in osteoarthritis. Rheumatology (Oxford),2020,59(10): 2681–2694. DOI: 10.1093/rheumatology/keaa248
[35] WANG Q, LEPUS C M, RAGHU H, et al. IgE-mediated mast cell activation promotes inflammation and cartilage destruction in osteoarthritis. Elife,2019,8: e39905. DOI: 10.7554/eLife.39905
[36] STAMBOUGH J B, CURTIN B M, ODUM S M, et al. Does change in ESR and CRP guide the timing of two-stage arthroplasty reimplantation? Clin Orthop Relat Res,2019,477(2): 364–371. DOI: 10.1097/01.blo.0000533618.31937.45
[37] KRISHNA A, GARG S, GUPTA S, et al. C-reactive protein (CRP) and erythrocyte sedimentation rate (ESR) trends following total hip and knee arthroplasties in an Indian population--a prospective study. Malays Orthop J,2021,15(2): 143–150. DOI: 10.5704/MOJ.2107.021
[38] 鲍丙溪, 刘健, 万磊, 等. 骨关节炎患者免疫炎症关键蛋白表达谱变化及中医药的干预研究. 中国免疫学杂志,2021,37(11): 1313–1318. DOI: 10.3969/j.issn.1000-484X.2021.11.007 [39] GRONWALL C, LILJEFORS L, BANG H, et al. A comprehensive evaluation of the relationship between different IgG and IgA anti-modified protein autoantibodies in rheumatoid arthritis. Front Immunol,2021,12: 627986. DOI: 10.3389/fimmu.2021.627986
[40] JONES K, SAVULESCU A F, BROMBACHER F, et al. Immunoglobulin M in health and diseases: how far have we come and what next? Front Immunol,2020,11: 595535. DOI: 10.3389/fimmu.2020.595535
-
期刊类型引用(1)
1. 周巧,刘健,朱艳,汪元,王桂珍,齐亚军,胡月迪. 生物信息学和机器学习策略识别骨关节炎炎性衰老生物标志物与临床验证. 四川大学学报(医学版). 2024(02): 279-289 . 百度学术
其他类型引用(1)
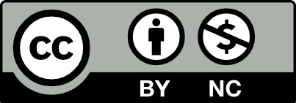
开放获取 本文遵循知识共享署名—非商业性使用4.0国际许可协议(CC BY-NC 4.0),允许第三方对本刊发表的论文自由共享(即在任何媒介以任何形式复制、发行原文)、演绎(即修改、转换或以原文为基础进行创作),必须给出适当的署名,提供指向本文许可协议的链接,同时标明是否对原文作了修改;不得将本文用于商业目的。CC BY-NC 4.0许可协议详情请访问 https://creativecommons.org/licenses/by-nc/4.0