改进型Unet网络在脑CT图像出血区域识别与分割中的应用
Application of Improved Unet Network in the Recognition and Segmentation of Hemorrhage Regions in Brain CT Images
-
摘要:目的 探讨改进型Unet网络技术对脑CT图像出血区域识别与分割的性能及其应用价值。方法 回顾性纳入476份自发性脑出血(spontaneous intracerebral hemorrhage, SICH)患者的脑CT图像,采用基于改进型Unet网络对患者脑CT图像出血区域进行识别和分割,由临床医生手动标注出血区域的影像数据,通过随机排序后,选取来自106例患者的430份数据进入训练集,11例患者的46份数据进入测试集,实验数据集通过数据增强后,经过网络训练和模型测试,以检验分割性能,并将分割结果与Unet网络(Base)、FCN-8s和Unet++网络进行对比。结果 改进型Unet网络对脑CT图像出血区域的分割中,相似性系数(Dice)、正向预测系数(PPV)、灵敏度系数(SC)三项评价指标分别达到0.8738、0.9011和0.8648,相较于FCN-8s网络分别提升8.80%、7.14%和8.96%,相较于Unet网络(Base)分别提升4.56%、4.44%和4.15%,同时优于Unet++网络的分割性能。结论 本文提出的基于Unet网络的改进方法对脑CT出血区域的识别和分割具有良好的性能,是一种脑CT出血区域识别和分割的适用方法,对于辅助临床决策和预防早期血肿扩张具有潜在应用价值。Abstract:Objective To examine the performance and application value of improved Unet network technology in the recognition and segmentation of hemorrhage regions in brain CT images.Methods A total of 476 brain CT images of patients with spontaneous intracerebral hemorrhage (SICH) were retrospectively included. The improved Unet network was used to identify and segment the hemorrhage regions in the patients’ brain CT images. The CT imaging data of the hemorrhage regions were manually labelled by clinicians. After randomized sorting, 430 data sets from 106 patients were selected for inclusion in the training set and 46 data sets from 11 patients were included in the test set. After data enhancement, the experimental data set underwent network training and model testing in order to assess the segmentation performance. The segmentation results were compared with the those of the Unet network (Base), FCN-8s network and Unet++ network.Results In the segmentation of brain CT image hemorrhage region with the improved Unet network, the three evaluation indicators of Dice similarity coefficient, positive predictive value (PPV), and sensitivity coefficient (SC) reached 0.8738, 0.9011 and 0.8648, respectively, increasing by 8.80%, 7.14% and 8.96%, respectively, compared with those of FCN-8s, and increasing by 4.56%, 4.44% and 4.15%, respectively, compared with those of Unet network (Base). The improved Unet network also showed better segmentation performance than that of Unet++ network.Conclusion The improved method based on Unet network proposed in this report displayed good performance in the recognition and segmentation of hemorrhage regions in brain CT images, and is an appropriate method for the recognition and segmentation of hemorrhage regions in brain CT images, showing potential application value for assisting clinical decision-making and preventing early hematoma expansion.
© 2022 《四川大学学报(医学版)》编辑部 版权所有
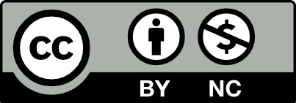
开放获取 本文遵循知识共享署名—非商业性使用4.0国际许可协议(CC BY-NC 4.0),允许第三方对本刊发表的论文自由共享(即在任何媒介以任何形式复制、发行原文)、演绎(即修改、转换或以原文为基础进行创作),必须给出适当的署名,提供指向本文许可协议的链接,同时标明是否对原文作了修改;不得将本文用于商业目的。CC BY-NC 4.0许可协议详情请访问 https://creativecommons.org/licenses/by-nc/4.0