Predicting Intensive Care Unit Mortality in Patients With Heart Failure Combined With Acute Kidney Injury Using an Interpretable Machine Learning Model: A Retrospective Cohort Study
-
摘要:目的
开发一种可解释的机器学习模型,以提高心力衰竭(heart failure, HF)合并急性肾损伤(acute kidney injury, AKI)患者短期死亡率的早期预测准确性。
方法本回顾性队列研究利用了基于电子病历的公开大型数据库重症医学信息数据库MIMIC-Ⅳ(Medical Information Mart for Intensive Care Ⅳ, 版本2.0)。提取了患者入住ICU最初24 h的数据,并将其分为训练集(80%)和验证集(20%)。利用沙普利可加性解释(Shapley additive explanation, SHAP)方法解释极端梯度提升(XGBoost)模型的工作原理,并识别关键的预后因素。使用曲线下面积(area under the curve, AUC)指标评估XGBoost模型的预测能力,并与3种其他机器学习模型进行比较,其解释性通过SHAP方法得到增强。
结果研究包括
8028 名合并AKI的HF患者。XGBoost模型的表现优于其他模型,达到了0.93的AUC〔95%置信区间(confidence interval, CI) 0.78~0.94,准确度=0.89〕,而神经网络的表现最差(AUC=0.79,95%CI 0.77~0.82,准确度=0.82)。决策曲线分析显示XGBoost模型在9%至80%的阈值概率内具有更高的净收益。SHAP分析识别了前20个预测因素,年龄以及格拉斯哥昏迷评分被识别为重要的因素,SHAP平均值分别为1.29和1.24。结论本研究开发的可解释模型提高了预测ICU内HF患者并发AKI的死亡风险的能力。这个模型不仅有助于制定有效的治疗计划,还能优化资源分配。
Abstract:ObjectiveHeart failure (HF) complicated by acute kidney injury (AKI) significantly impacts patient outcomes, and it is crucial to make early predictions of short-term mortality. This study is focused on developing an interpretable machine learning model to enhance early prediction accuracy in such clinical scenarios.
MethodsThis retrospective cohort study utilized data from the Medical Information Mart for Intensive Care Ⅳ (MIMIC-Ⅳ, version 2.0) database. Data from the first 24 hours after admission to the ICU were extracted and divided into a training set (70%) and a validation set (30%). We utilized the SHapley Additive exPlanation (SHAP) method to interpret the workings of an extreme gradient boosting (XGBoost) model and identify key prognostic factors. The XGBoost model's predictive ability was evaluated against three other machine learning models using the area under the curve (AUC) metric, and its interpretation was enhanced using the SHAP method.
ResultsThe study included
8028 patients with HF complicated by AKI. The XGBoost model outperformed the other models, achieving an AUC of 0.93 (95% confidence interval [CI]: 0.78-0.94; accuracy = 0.89), while neural network model showed the worst performance (AUC = 0.79, 95% CI: 0.77-0.82; accuracy = 0.82). Decision curve analysis showed the superior net benefit of the XGBoost model within the 9% to 60% threshold probabilities. SHAP analysis was performed to identify the top 20 predictors, with age (mean SHAP value 1.29) and Glasgow Coma Scale score (mean SHAP value 1.24) emerging as significant factors.ConclusionsOur interpretable model offers an enhanced ability to predict mortality risk in HF patients with AKI in ICUs. This model can be used to assist in formulating effective treatment plans and optimizing resource allocation.
-
Keywords:
- Acute kidney injury /
- Heart failure /
- Machine learning /
- Predictive model /
- MIMIC
-
心力衰竭(heart failure, HF)是一种严重危及生命的综合临床病症,其发病率高、死亡率高,在全球范围内广泛存在,影响着全球超过
6400 万人[1],在中国65岁以上的人群中患病率超过10%[2]。重症HF的病情进展迅速,短时间内可导致多器官衰竭、心肺循环障碍、急性肾损伤(acute kidney injury, AKI)等并发症[3]。心脏和肾脏之间常常相互影响,其中一个器官的损伤可能导致另一个器官的损伤,无法进行代偿,从而导致心脏和肾脏同时受损,即心肾综合征(cardiorenal syndromes, CRS)[4]。Ⅰ型心肾综合征是指急性心脏疾病患者发生AKI,有研究显示, 24%~45%的急性心力衰竭(acute heart failure, AHF)患者会发生AKI,尤其是在重症监护病房(intensive care unit, ICU)的患者中,AKI往往预示着较高的短期和长期死亡率[5-6]。目前,HF并发AKI的具体机制尚不清楚。传统理论认为,HF会导致心输出量不足,从而使肾脏灌注减少,引发肾缺血,进而导致AKI。同时,机体通过激活交感神经、肾素-血管紧张素-醛固酮系统等机制,使肾小球滤过率降低,增加钠和水的吸收,导致水钠潴留,进而加重心衰,形成恶性循环[7-8]。二者相互作用,相互影响,不仅会加重患者的病情,延长患者的住院时间,还会增加患者的死亡风险。尽管对症支持治疗可以改善患者的病情,但仍有部分患者出现多器官功能衰竭、死亡[9]。因此,早期有效预测重症HF合并AKI的患者预后十分必要,以便临床及时调整治疗方案,避免延误治疗时间。不幸的是,针对这一问题的研究相对较少。
近年来,人工智能被广泛用于探索许多疾病的早期预警指标。鉴于机器学习算法捕捉非线性关系的强大特性,越来越多的研究者并不主张使用传统的疾病严重性分类系统,如序贯器官衰竭评估(sequential organ failure assessment,SOFA)、急性生理和慢性健康评估 Ⅱ或简化急性生理评分Ⅱ,而更倾向于基于机器学习的新预测模型来为患者的治疗提供支持[10-13]。尽管大量预测模型在研究中展示了良好的性能,但它们在临床设置中的应用证据和用于疾病预后辅助的可解释性风险预测模型仍然研究有限。
本研究的目的是开发一个可解释的模型,使用开放的重症监护数据库MIMIC-Ⅳ预测ICU中HF合并AKI患者的死亡风险。此外,使用沙普利可加性解释(Shapley additive explanation, SHAP)方法来解释极端梯度提升(XGBoost)模型并探索HF合并AKI的预后因素。
1. 资料与方法
1.1 数据来源
本研究使用的数据源自MIMIC-Ⅳ数据库,这是一个由麻省理工学院提供的公开且免费的综合性重症监护数据库。它涵盖了2008–2019年,在波士顿的贝斯以色列女执事医院接受ICU治疗的超过
70000 名患者的信息。本研究已获得了使用该数据库的批准(LXY,认证号12059504)。1.2 伦理考虑
MIMIC-Ⅳ数据库的建立已获得麻省理工学院和贝斯以色列女执事医疗中心的机构审查委员会批准。所有纳入的患者均进行了脱敏处理以保护其隐私。本研究为回顾性观察研究,并基于《流行病学观察研究报告加强指南》(STROBE)进行了报告。
1.3 研究人群纳入
符合条件的AKI患者为18岁以上且住院时间超过48 h的患者。排除标准:①个人数据缺失超过5%;②基线值超出中位数±1.5倍四分位距;③在入ICU前诊断为终末期肾病。根据改善全球肾脏病预后组织(Kidney Disease: Improving Global Outcomes, KDIGO)指南[14]诊断AKI,该指南规定了血清肌酐(serum creatinine, SCr)水平和尿量变化。第一阶段定义为在前7 d内SCr增加≥1.5倍基线值或在48 h内SCr增加0.3 mg/dL,或6 h尿量<0.5 mL/(kg·h)。第二阶段的特点是SCr增加≥2.0倍基线或12 h尿量<0.5 mL/(kg·h)。第三阶段的特点是SCr增加≥3.0倍基线,SCr ≥4.0 mg/dL,开始肾脏替代治疗(RRT),或24 h尿量<0.3 mL/(kg·h)。患者在ICU入院前6 h至入院后48 h内根据KDIGO指南被诊断为AKI。基线SCr指的是在AKI诊断前7 d内或48 h内的最低值。
1.4 数据提取
结局定义为患者入院后30 d的全因死亡率。患者数据是通过使用结构化查询语言(SQL)和PostgreSQL工具(版本15.1)从MIMIC-Ⅳ数据库中提取的。提取的数据包含患者识别信息、临床参数、实验室参数、共患病和评分系统。临床参数包括年龄、种族、性别、心率、呼吸频率、收缩压(systolic blood pressure, SBP)、舒张压(diastolic blood pressure, DBP)、平均动脉压(mean arterial pressure, MAP)、经皮氧饱和度(peripheral oxygen saturation, SpO2)、使用加压素、使用呼吸机以及肾脏替代治疗(renal replacement therapy, RRT)。实验室参数包括葡萄糖、氯化物、阴离子间隙、碳酸氢盐、乳酸、血尿素氮(blood urea nitrogen, BUN)水平、SCr水平、尿量、钾、钠、单核细胞、淋巴细胞、血小板、红细胞(red blood cells, RBC)、红细胞分布宽度(red cell distribution width, RDW)、平均红细胞体积(mean corpuscular volume, MCV)、国际标准化比率(international normalized ratio, INR)和白细胞(white blood cells, WBC)。本研究还根据国际疾病分类(ICD-9/10)代码定义提取了以下共患病情况:高血压、糖尿病、冠状动脉疾病、慢性阻塞性肺病(chronic obstructive pulmonary disease, COPD)、恶性肿瘤、血液病、心房颤动、肝病、休克、脓毒症。评分系统包括SOFA得分和格拉斯哥昏迷量表(GCS)得分,每个患者均进行了计算。对于有多次ICU入院的患者,只有首次入院的数据被考虑在内。本研究使用患者在ICU入院前6 h和入院后48 h内的首次测量值作为基线数据。此外,为避免过拟合,本研究使用逐步选择模型进行变量的选择和筛选。
1.5 缺失值处理
本研究在排除了数据丢失超过5%的患者后,采用了多重插补法来处理缺失数据。所有选定的变量缺失值均少于30%。数据被假定为随机缺失,并使用R语言(v4.2.2)的“mice”软件包以分类,通过回归树方法进行插补。具体设定为插补次数为 5 次,最大迭代次数为 5 次,以确保生成合理的插补。
1.6 机器学习模型解释工具SHAP
本研究使用SHAP进行预测模型的解释。SHAP是一种用于精确计算每个特征对最终预测结果的贡献和影响的方法[15]。SHAP值能够明确展示每个预测变量对目标结局的正贡献或负贡献程度。使用SHAP Python软件包的函数来评估剩余特征对预测得分的影响,该软件包采用了博弈论方法来评估每个特征的重要性。在每次循环中,本研究使用了递归特征消除方法,影响预测最小的特征被消除,从而生成一个简化的模型。
1.7 统计学方法
所有的统计分析和计算工作均通过R语言和Python 3.1.2完成。对于连续性变量,使用均值和标准差来描述;对于非正态分布的变量,则使用四分位数范围和中位数来描述,而在两组间进行比较时,应用了Wilcoxon秩和检验。对于分类变量,则以百分比和计数形式展示,使用卡方检验或(当预期频率低于10时)Fisher精确检验来分析组间的差异。本研究将最终纳入研究的患者分为训练集(80%)和验证集(20%)。4种机器学习模型——XGBoost、逻辑回归(logistic regression, LR)、随机森林(random forest, RF)和神经网络(neural network, NN)被用于开发预测模型。XGBoost模型设置了树的数量
2000 ,树的最大深度12。随机森林模型设置了树的最大深度6,以及树的数量1000 。神经网络模型的超参数包括激活函数为tanh,最大迭代次数100000 ,隐藏层结构为(200,3),其他参数均为所使用版本的默认值。逻辑回归模型采用该版本的默认参数配置,核心包版本如下:scikit-learn 1.5.2,numpy 2.1.3,xgboost 2.1.3。每个模型的预测性通过接收者操作特征曲线下的面积(area under the curve, AUC)来评估。考虑本模型为临床模型,假阳性可能带来不必要的干预和治疗,因此本研究将预测的特异性固定在85%,计算此时的准确性、灵敏度、正预测值、负预测值和F1得分。此外,本研究执行了决策曲线分析(decision curve analysis, DCA),该分析通过计算在各种阈值概率下的净收益,用以衡量模型在实际决策过程中的应用价值。临床净效益被定义为需要进一步干预时疾病发生的最小概率[16]。2. 结果
2.1 患者特征
在MIMIC-Ⅳ中有
8028 例HF合并AKI患者中,总共7730 例被诊断为原发性HF的成年患者被包括在本研究的最终队列中。患者筛查过程如图1所示。数据集随机分为2个部分:数据集的80%(n=5864 )进行模型训练,数据集的20%(n=1466 )进行模型验证。通过逐步选择模型,本研究在5412例 患者的基础上产生了47个潜在预测因子,这些预测因素用于模型开发。在训练数据集中,30 d全因死亡率为20.15%(1034 /5131 ),测试数据集中的死亡率为20.38%(448/2199 )。表1展示了在训练集和测试集之间的预测变量比较。表 1 研究人群的基线数据Table 1. Baseline characteristics of the study populationCharacteristic Train set (n= 5864 )Validation set (n= 1466 )P Age/yr. 74.22±13.16 73.92±13.08 0.43 Sex/case (%) 0.18 Female 643 (43.90) 2693 (45.90)Male 823 (56.10) 3171 (54.10)Ethnicity/case (%) 0.57 Asian 21 (1.40) 130 (2.20) Black 128 (8.70) 495 (8.40) Latino 47 (3.20) 137 (2.30) Others 264 (18.00) 971 (16.60) White 1006 (68.60)4131 (70.40)Heart rate/min-1, M (P25, P75) 86.00 (75.00, 101.00) 86.00 (75.00, 101.00) 0.93 SBP/mmHg, M (P25, P75) 119.00 (103.00, 136.00) 118.00 (102.00, 135.00) 0.10 DBP/mmHg, M (P25, P75) 63.00 (53.00, 75.00) 63.00 (52.00, 75.00) 0.04 MBP/mmHg, M (P25, P75) 79.00 (69.00, 92.00) 78.00 (67.00, 90.00) 0.03 Respiratory rate/min-1, M (P25, P75) 19.00 (16.00, 23.75) 18.00 (16.00, 23.00) 0.08 Temperature/℃, M (P25, P75) 36.70 (36.33, 37.06) 36.67 (36.28, 37.00) 0.02 SpO2/%, M (P25, P75) 98.00 (95.00, 100.00) 98.00 (95.00, 100.00) 0.17 Hypertension/case (%) 799 (54.50) 3178 (54.20)0.86 Diabetes/case (%) 624 (42.60) 2407 (41.00)0.31 Coronary artery disease/case (%) 804 (54.80) 3145 (53.60)0.42 COPD/case (%) 266 (18.10) 941 (16.00) 0.06 Malignancy /case (%) 173 (11.80) 623 (10.60) 0.21 Liver disease/case (%) 188 (12.80) 760 (13.00) 0.92 Deficiency anemia/case (%) 736 (50.20) 2954 (50.40)0.93 Atrial fibrillation/case (%) 323 (22.00) 1189 (20.30)0.15 Shock/case (%) 341 (23.30) 1443 (24.60)0.30 Sepsis/case (%) 1020 (69.60)4089 (69.70)0.93 Anion gap/(mmol/L), M (P25, P75) 15.00 (12.00, 18.00) 15.00 (13.00, 18.00) 0.72 BUN/(mg/dL), M (P25, P75) 26.00 (18.00, 41.00) 27.00 (18.00, 42.00) 0.74 Serum creatinine/(mg/dL), M (P25, P75) 1.20 (0.90, 1.80) 1.20 (0.90, 1.80) 0.33 RBC/×1012 L-1, M (P25, P75) 3.65 (3.09, 4.23) 3.63 (3.06, 4.21) 0.50 RDW/%, M (P25, P75) 14.90 (13.90, 16.50) 14.90 (13.90, 16.40) 0.84 MCH/pg, M (P25, P75) 29.90 (28.13, 31.48) 30.00 (28.30, 31.50) 0.43 MCV/fL, M (P25, P75) 91.00 (87.00, 96.00) 91.00 (87.00, 96.00) 0.94 Hemoglobin/(g/dL), M (P25, P75) 10.70 (9.10, 12.40) 10.70 (9.10, 12.40) 0.79 Hematocrit (M [P25, P75]) 33.20 (28.10, 38.20) 32.80 (28.00, 38.00) 0.52 Platelets/×109 L-1, M (P25, P75) 202.00 (146.00, 270.00) 200.00 (148.00, 268.00) 0.66 PTT/s, M (P25, P75) 32.70 (27.90, 42.38) 32.70 (28.00, 41.82) 0.96 Potassium/(mmol/L), M (P25, P75) 4.25 (3.80, 4.80) 4.30 (3.90, 4.80) 0.93 Sodium/(mmol/L), M (P25, P75) 139.00 (136.00, 141.00) 139.00 (135.00, 141.00) 0.48 Calcium/(mg/dL), M (P25, P75) 8.40 (8.00, 8.90) 8.50 (8.00, 8.90) 0.22 Chloride/(mmol/L), M (P25, P75) 103.00 (99.00, 107.00) 103.00 (99.00, 107.00) 0.93 Bicarbonate/(mmol/L), M (P25, P75) 24.00 (21.00, 27.00) 23.00 (20.00, 27.00) 0.13 SOFA score (M [P25, P75]) 6.00 (4.00, 9.00) 6.00 (4.00, 9.00) 0.63 GCS score (M [P25, P75]) 14.00 (10.00, 15.00) 14.00 (10.00, 14.00) 0.35 AKI KDIGO stage/case (%) 0.99 Stage 1 356 (24.30) 1434 (24.50)Stage 2 745 (50.80) 2977 (50.80)Stage 3 365 (24.90) 1453 (24.80)Vasoactive use/case (%) 826 (56.30) 3402 (58.00)0.26 Ventilator use/case (%) 808 (55.10) 3267 (55.70)0.70 SBP: systolic blood pressure; DBP: diastolic blood pressure; MBP: mean blood pressure; SpO2: percutaneous oxygen saturation; COPD: chronic obstructive pulmonary disease; BUN: blood urea nitrogen; RBC: red blood count; RDW: red cell distribution width; MCH: mean corpuscular hemoglobin; MCV: mean corpuscular volume; PTT: partial thromboplastin time; SOFA: Sequential Organ Failure Assessment; GCS: Glasgow Coma Scale; KDIGO: Kidney Disease Improving Global Outcomes. 1 mmHg = 0.133 kPa. 2.2 模型建立和评估
在训练数据集中建立了XGBoost、LR、RF和NN模型,测试数据集获得的AUC分别为0.93、0.80、0.79和0.84(表2和图2)。相比之下,四个模型中 XGBoost 的预测性能最高〔AUC=0.93,95%置信区间(confidence interval, CI)0.78~0.94〕而NN的泛化能力最差(AUC=0.79,95%CI 0.77~0.82)。对测试数据集中的四个机器学习模型进行DCA,以比较最佳模型和临床决策替代方法的临床净效益。
表 2 四种模型的预测表现Table 2. Performance of each model for predictionModel AUC Sensitivity Specificity F1
scoreAccuracy PPV NPV LR 0.80 (0.77-0.84) 0.30 0.96 0.80 0.82 0.68 0.84 RF 0.84 (0.81-0.87) 0.07 1.00 0.73 0.81 0.96 0.80 NN 0.79 (0.77-0.82) 0.39 0.94 0.81 0.82 0.62 0.85 XGBoost 0.91 (0.78-0.94) 0.60 0.97 0.88 0.89 0.82 0.90 AUC: area under the curve; PPV: positive predictive value; NPV: negative predictive value; LR: logistic regression; RF: random forest; NN: neural network. 图3展示了在不同阈值概率条件下的净效益分析,其中,浅灰色曲线假定对所有患者采取治疗措施,黑色曲线则假定没有患者被治疗。鉴于研究群体的多样性,任一采用机器学习方法构建的四个模型提出的治疗方案都明显优于全部治疗或不治疗的标准方案。特别地,XGBoost模型在9%到80%的阈值范围内实现的净效益高于其他模型。
2.3 使用SHAP方法解释XGBoost模型
SHAP算法用于获取每个预测变量对XGBoost模型预测结果的重要性。变量重要性图按降序列出了最重要的变量(图4)。年龄的平均值在所有预测范围内具有最强的预测价值,其次是GCS评分、BUN、休克和RDW。此外,为了检测预测变量与目标结果的正相关和负相关关系,应用SHAP值来揭示死亡风险因素。如图5所示,水平位置显示该值的影响是否与较高或较低的预测相关,颜色显示该变量对于该观察结果是高(红色)还是低(蓝色);结果显示,平均年龄的增加具有积极的影响,并将预测推向死亡率,其SHAP平均值为1.29,而GCS评分的SHAP平均值为1.24,其增加具有负面影响,将预测推向生存。
2.4 SHAP个体力图
图6显示了存活和未存活的患者的个体力图。SHAP 值表示个体患者的预测相关特征以及每个特征对死亡率预测的贡献。粗体数字是概率预测值〔f(x)〕,而基值是在不向模型提供输入的情况下预测的值。f(x) 是每个观测值的对数优势比。红色特征(左侧)表示增加死亡风险的特征,蓝色特征(右侧)表示降低死亡风险的特征。箭头的长度用于可视化对预测的影响程度,箭头越长,效果越大。
为进一步剖析模型预测的不足,本文以一名实际未存活但模型预测存活的患者为例进行了详细分析。患者83岁,患有慢性阻塞性肺病、高血压、冠心病、肝病和贫血等多种慢性疾病,入院时伴有感染性休克,使用了升压药和机械通气,SOFA评分为5,实验室检查提示血小板计数低(82)、RDW升高(17.6)、血钙偏低(7.9)。SHAP值的个体力图显示,模型将GCS评分较高(12分)和部分正常实验室指标(如PTT正常)作为降低死亡风险的主要特征,而高龄、高RDW和低血小板等死亡风险增加的特征未被赋予足够权重。预测失败的原因可能包括:模型对慢性病和急性病程特征的交互效应低估,例如未能充分权衡慢性基础疾病和急性病情的复合作用,导致对患者综合风险的评估不足,缺乏动态病情变化数据,如SOFA评分的动态变化和血流动力学恶化趋势,这对于评估病情进展的严重性尤为重要。静态数据可能掩盖了患者病情在短期内急剧恶化的过程,例如血压持续下降、氧合指数恶化或器官功能恶化的加速趋势,而这些动态信息通常是病情转归的重要决定因素;对感染性休克治疗特征的风险贡献未能充分体现,例如升压药的长期使用可能提示循环功能的严重损害,而机械通气的应用可能暗示呼吸衰竭的严重程度,这些信息在模型中的影响被低估。这表明模型在处理慢性病合并急性病变的高龄患者时存在局限性,后续研究可通过增加动态特征和优化变量权重等方式进一步改进。
3. 讨论
在这项针对大型公共ICU数据库的回顾性队列研究中,我们开发并验证了四种机器学习算法,以预测HF合并AKI患者的死亡率。XGBoost模型的性能超过了LR、RF和NN。这是因为XGBoost模型能够高效灵活地处理缺失数据,并能够捕捉数据中的复杂关系,将弱预测模型集合起来构建准确的预测模型[17]。SHAP方法被用来解释XGBoost模型,确保了模型的性能和临床可解释性。这将帮助医生更好地理解模型的决策过程,并促进预测结果的使用。此外,为了避免无效的临床干预,本研究关注的DCA相关阈值概率范围在9%到60%之间,在此期间XGBoost表现更佳。研究表明XGBoost已广泛用于预测患者的住院死亡率,并可能协助临床医生进行决策[18-20]。虽然本研究中XGBoost展现出了优秀的预测能力,阳性预测值达到0.82,但由于缺乏更多的外部验证其实用性,XGBoost模型距离实际应用于临床还有一定的距离。
使用SHAP解释XGBoost模型,本研究识别了一些与HF合并AKI患者住院死亡率相关的重要变量。在本研究中,年龄及GCS评分被认为是最重要的预测变量。年龄是许多疾病的已知风险因素,包括心力衰竭和肾脏疾病。一项系统综述纳入13项研究并分析了15个危险因素,其中年龄是HF合并AKI患者的预测因素[8]。在一项HF合并AKI预测风险评分系统中,年龄被纳入风险评分[21],可见年龄是HF合并AKI重要的预测变量。此外,年龄增大常常与并存疾病相关,比如抑郁症、极端体质量指数、虚弱等,这些并存疾病可能使HF和AKI的管理复杂化,导致更高的死亡风险[22]。GCS是一个评估人的意识水平的神经学评分系统,尤其是在头部受伤后。它通过刺激反应来评估患者的语言、运动和睁眼情况。研究表明,GCS评分是重症患者死亡率的准确预测指标,降低的GCS评分与危重患者的死亡率增加相关[23- 24]。在本研究中,较低的GCS评分与HF合并AKI的患者较高的死亡率相关,这表明HF合并AKI的患者可能有严重的脑功能障碍,可能是由于心脏衰竭导致的血液灌注不良,影响大脑的氧气供应,在这种情况下患者死亡风险更高。这也很可能是因为这些个体的疾病严重程度较高。由于缺乏外部验证队列,需要进一步研究来探索这种SHAP方法的适用性。
本研究存在一定的局限性。首先,本研究数据源自公开可获取的数据库,其中一些变量存在缺失情况。例如,本研究原计划包括更多可能影响院内死亡率的预测变量,如肾损伤分子-1、乳酸和B型钠利尿肽;然而,由于这些变量的缺失率超过了70%,因此未能在研究中使用。其次,白人在所有病例中占据了70%的比例,这导致了本研究建立的模型在其他人群中的适用性仍然存在不确定性。第三,本研究死亡率预测模型基于每次ICU入院后的24 h内可获得的数据,这可能会忽略后续数据的影响,从而影响了预测准确性,并在一定程度上引入了混杂因素。第四,由于缺乏外部验证队列,本研究开发的XGBoost模型在临床实践中的适用性可能不如预期高效。
* * *
作者贡献声明 罗欣瑶负责论文构思、数据审编、正式分析、研究方法、验证、可视化、初稿写作和审读与编辑写作,万丁源负责论文构思、数据审编、正式分析、验证和可视化,王轲负责数据审编、正式分析、初稿写作和审读与编辑写作,苏白海负责经费获取、提供资源、监督指导、初稿写作和审读与编辑写作。所有作者已经同意将文章提交给本刊,且对将要发表的版本进行最终定稿,并同意对工作的所有方面负责。
Author Contribution LUO Xinyao is responsible for conceptualization, data curation, formal analysis, methodology, validation, visualization, writing--original draft, and writing--review and editing.WAN Dingyuan is responsible for conceptualization, data curation, formal analysis, validation, and visualization. WANG Ke is responsible for data curation, formal analysis, writing--original draft, and writing--review and editing. SU Baihai is responsible for funding acquisition, resources, supervision, writing--original draft, and writing--review and editing. All authors consented to the submission of the article to the Journal. All authors approved the final version to be published and agreed to take responsibility for all aspects of the work.
利益冲突 所有作者均声明不存在利益冲突
Declaration of Conflicting Interests All authors declare no competing interests.
-
表 1 研究人群的基线数据
Table 1 Baseline characteristics of the study population
Characteristic Train set (n= 5864 )Validation set (n= 1466 )P Age/yr. 74.22±13.16 73.92±13.08 0.43 Sex/case (%) 0.18 Female 643 (43.90) 2693 (45.90)Male 823 (56.10) 3171 (54.10)Ethnicity/case (%) 0.57 Asian 21 (1.40) 130 (2.20) Black 128 (8.70) 495 (8.40) Latino 47 (3.20) 137 (2.30) Others 264 (18.00) 971 (16.60) White 1006 (68.60)4131 (70.40)Heart rate/min-1, M (P25, P75) 86.00 (75.00, 101.00) 86.00 (75.00, 101.00) 0.93 SBP/mmHg, M (P25, P75) 119.00 (103.00, 136.00) 118.00 (102.00, 135.00) 0.10 DBP/mmHg, M (P25, P75) 63.00 (53.00, 75.00) 63.00 (52.00, 75.00) 0.04 MBP/mmHg, M (P25, P75) 79.00 (69.00, 92.00) 78.00 (67.00, 90.00) 0.03 Respiratory rate/min-1, M (P25, P75) 19.00 (16.00, 23.75) 18.00 (16.00, 23.00) 0.08 Temperature/℃, M (P25, P75) 36.70 (36.33, 37.06) 36.67 (36.28, 37.00) 0.02 SpO2/%, M (P25, P75) 98.00 (95.00, 100.00) 98.00 (95.00, 100.00) 0.17 Hypertension/case (%) 799 (54.50) 3178 (54.20)0.86 Diabetes/case (%) 624 (42.60) 2407 (41.00)0.31 Coronary artery disease/case (%) 804 (54.80) 3145 (53.60)0.42 COPD/case (%) 266 (18.10) 941 (16.00) 0.06 Malignancy /case (%) 173 (11.80) 623 (10.60) 0.21 Liver disease/case (%) 188 (12.80) 760 (13.00) 0.92 Deficiency anemia/case (%) 736 (50.20) 2954 (50.40)0.93 Atrial fibrillation/case (%) 323 (22.00) 1189 (20.30)0.15 Shock/case (%) 341 (23.30) 1443 (24.60)0.30 Sepsis/case (%) 1020 (69.60)4089 (69.70)0.93 Anion gap/(mmol/L), M (P25, P75) 15.00 (12.00, 18.00) 15.00 (13.00, 18.00) 0.72 BUN/(mg/dL), M (P25, P75) 26.00 (18.00, 41.00) 27.00 (18.00, 42.00) 0.74 Serum creatinine/(mg/dL), M (P25, P75) 1.20 (0.90, 1.80) 1.20 (0.90, 1.80) 0.33 RBC/×1012 L-1, M (P25, P75) 3.65 (3.09, 4.23) 3.63 (3.06, 4.21) 0.50 RDW/%, M (P25, P75) 14.90 (13.90, 16.50) 14.90 (13.90, 16.40) 0.84 MCH/pg, M (P25, P75) 29.90 (28.13, 31.48) 30.00 (28.30, 31.50) 0.43 MCV/fL, M (P25, P75) 91.00 (87.00, 96.00) 91.00 (87.00, 96.00) 0.94 Hemoglobin/(g/dL), M (P25, P75) 10.70 (9.10, 12.40) 10.70 (9.10, 12.40) 0.79 Hematocrit (M [P25, P75]) 33.20 (28.10, 38.20) 32.80 (28.00, 38.00) 0.52 Platelets/×109 L-1, M (P25, P75) 202.00 (146.00, 270.00) 200.00 (148.00, 268.00) 0.66 PTT/s, M (P25, P75) 32.70 (27.90, 42.38) 32.70 (28.00, 41.82) 0.96 Potassium/(mmol/L), M (P25, P75) 4.25 (3.80, 4.80) 4.30 (3.90, 4.80) 0.93 Sodium/(mmol/L), M (P25, P75) 139.00 (136.00, 141.00) 139.00 (135.00, 141.00) 0.48 Calcium/(mg/dL), M (P25, P75) 8.40 (8.00, 8.90) 8.50 (8.00, 8.90) 0.22 Chloride/(mmol/L), M (P25, P75) 103.00 (99.00, 107.00) 103.00 (99.00, 107.00) 0.93 Bicarbonate/(mmol/L), M (P25, P75) 24.00 (21.00, 27.00) 23.00 (20.00, 27.00) 0.13 SOFA score (M [P25, P75]) 6.00 (4.00, 9.00) 6.00 (4.00, 9.00) 0.63 GCS score (M [P25, P75]) 14.00 (10.00, 15.00) 14.00 (10.00, 14.00) 0.35 AKI KDIGO stage/case (%) 0.99 Stage 1 356 (24.30) 1434 (24.50)Stage 2 745 (50.80) 2977 (50.80)Stage 3 365 (24.90) 1453 (24.80)Vasoactive use/case (%) 826 (56.30) 3402 (58.00)0.26 Ventilator use/case (%) 808 (55.10) 3267 (55.70)0.70 SBP: systolic blood pressure; DBP: diastolic blood pressure; MBP: mean blood pressure; SpO2: percutaneous oxygen saturation; COPD: chronic obstructive pulmonary disease; BUN: blood urea nitrogen; RBC: red blood count; RDW: red cell distribution width; MCH: mean corpuscular hemoglobin; MCV: mean corpuscular volume; PTT: partial thromboplastin time; SOFA: Sequential Organ Failure Assessment; GCS: Glasgow Coma Scale; KDIGO: Kidney Disease Improving Global Outcomes. 1 mmHg = 0.133 kPa. 表 2 四种模型的预测表现
Table 2 Performance of each model for prediction
Model AUC Sensitivity Specificity F1
scoreAccuracy PPV NPV LR 0.80 (0.77-0.84) 0.30 0.96 0.80 0.82 0.68 0.84 RF 0.84 (0.81-0.87) 0.07 1.00 0.73 0.81 0.96 0.80 NN 0.79 (0.77-0.82) 0.39 0.94 0.81 0.82 0.62 0.85 XGBoost 0.91 (0.78-0.94) 0.60 0.97 0.88 0.89 0.82 0.90 AUC: area under the curve; PPV: positive predictive value; NPV: negative predictive value; LR: logistic regression; RF: random forest; NN: neural network. -
[1] SAVARESE G, BECHER P M, LUND L H, et al. Global burden of heart failure: a comprehensive and updated review of epidemiology. Cardiovasc Res, 2023, 118(17): 3272-87. doi: 10.1093/cvr/cvac013.
[2] WANG H, CHAI K, DU M, et al. Prevalence and incidence of heart failure among urban patients in china: a national population-based analysis. Circ Heart Fail, 2021, 14(10): e008406. doi: 10.1161/CIRCHEARTFAILURE.121.008406.
[3] PACKER D L, PICCINI J P, MONAHAN K H, et al. Ablation versus drug therapy for atrial fibrillation in heart failure: results from the CABANA trial. Circulation, 2021, 143(14): 1377-1390. doi: 10.1161/CIRCULATIONAHA.120.050991.
[4] RONCO C, MCCULLOUGH P, ANKER S D, et al. Cardio-renal syndromes: report from the consensus conference of the acute dialysis quality initiative. Eur Heart J, 2010, 31(6): 703-711. doi: 10.1093/eurheartj/ehp507.
[5] RONCO C, CICOIRA M, MCCULLOUGH P A. Cardiorenal syndrome type 1: pathophysiological crosstalk leading to combined heart and kidney dysfunction in the setting of acutely decompensated heart failure. J Am Coll Cardiol, 2012, 60(12): 1031-1042. doi: 10.1016/j.jacc.2012.01.077.
[6] VANDENBERGHE W, GEVAERT S, KELLUM J A, et al. Acute kidney injury in cardiorenal syndrome type 1 patients: a systematic review and meta-analysis. Cardiorenal Med, 2016, 6(2): 116-128. doi: 10.1159/000442300.
[7] MéNDEZ A B, AZANCOT M A, OLIVELLA A, et al. New aspects in cardiorenal syndrome and HFpEF. Clin Kidney J, 2022, 15(10): 1807-1815. doi: 10.1093/ckj/sfac133.
[8] RU S C, LV S B, LI Z J. Incidence, mortality, and predictors of acute kidney injury in patients with heart failure: a systematic review. ESC Heart Fail, 2023, 10(6): 3237-3249. doi: 10.1002/ehf2.14520.
[9] 王莹, 骆继业, 周艺. NT-proBNP、ETX及HBP水平对重症心力衰竭合并急性肾损伤患者预后的预测价值. 中国现代医学杂志, 2023, 33(2): 94-100. doi: 10.3969/j.issn.1005-8982.2023.02.016. WANG Y, LUO J Y, ZHOU Y. Prognostic value of NT-proBNP, ETX and HBP levels in patients with severe heart failure complicated with acute kidney injury. Chin J Mod Med, 2023, 33(2): 94-100. doi: 10.3969/j.issn.1005-8982.2023.02.016.
[10] TSENG P Y, CHEN Y T, WANG C H, et al. Prediction of the development of acute kidney injury following cardiac surgery by machine learning. Crit Care, 2020, 24(1): 478. doi: 10.1186/s13054-020-03179-9.
[11] DONG J, FENG T, THAPA-CHHETRY B, et al. Machine learning model for early prediction of acute kidney injury (AKI) in pediatric critical care. Crit care, 2021, 25(1): 288. doi: 10.1186/s13054-021-03724-0.
[12] YUE S, LI S, HUANG X, et al. Machine learning for the prediction of acute kidney injury in patients with sepsis. J Transl Med, 2022, 20(1): 215. doi: 10.1186/s12967-022-03364-0.
[13] FAN Z, JIANG J, XIAO C, et al. Construction and validation of prognostic models in critically Ill patients with sepsis-associated acute kidney injury: interpretable machine learning approach. J Transl Med, 2023, 21(1): 406. doi: 10.1186/s12967-023-04205-4.
[14] LAMEIRE N H, LEVIN A, KELLUM J A, et al. Harmonizing acute and chronic kidney disease definition and classification: report of a Kidney Disease: Improving Global Outcomes (KDIGO) Consensus Conference. Kidney Int, 2021, 100(3): 516-526. doi: 10.1016/j.kint.2021.06.028.
[15] LUNDBERG S M, LEE S I. A unified approach to interpreting model predictions. Proceedings of the 31st International Conference on Neural Information Processing Systems. Long Beach, California, USA: Curran Associates Inc, 2017: 4768-4777.
[16] LEE C, LIGHT A, ALAA A, et al. Application of a novel machine learning framework for predicting non-metastatic prostate cancer-specific mortality in men using the Surveillance, Epidemiology, and End Results (SEER) database. Lancet Digit Health, 2021, 3(3): e158-e165. doi: 10.1016/S2589-7500(20)30314-9.
[17] YUAN K C, TSAI L W, LEE K H, et al. The development an artificial intelligence algorithm for early sepsis diagnosis in the intensive care unit. Int J Med Inform, 2020, 141: 104176. doi: 10.1016/j.ijmedinf.2020.104176.
[18] LI J, LIU S, HU Y, et al. Predicting mortality in intensive care unit patients with heart failure using an interpretable machine learning model: retrospective cohort study. J Med Internet Res, 2022, 24(8): e38082. doi: 10.2196/38082.
[19] ZHANG Z, HO K M, HONG Y. Machine learning for the prediction of volume responsiveness in patients with oliguric acute kidney injury in critical care. Crit care, 2019, 23(1): 112. doi: 10.1186/s13054-019-2411-z.
[20] PAN P, LI Y, XIAO Y, et al. Prognostic assessment of COVID-19 in the intensive care unit by machine learning methods: model development and validation. J Med Internet Res, 2020, 22(11): e23128. doi: 10.2196/23128.
[21] ZHOU L Z, YANG X B, GUAN Y, et al. Development and validation of a risk score for prediction of acute kidney injury in patients with acute decompensated heart failure: a prospective cohort study in China. J Am Heart Assoc, 2016, 5(11): e004035. doi: 10.1161/JAHA.116.004035.
[22] REGAN J A, KITZMAN D W, LEIFER E S, et al. Impact of age on comorbidities and outcomes in heart failure with reduced ejection fraction. JACC Heart Fail, 2019, 7(12): 1056-1065. doi: 10.1016/j.jchf.2019.09.004.
[23] BHAGWANJEE S, PARUK F, MOODLEY J, et al. Intensive care unit morbidity and mortality from eclampsia: an evaluation of the Acute Physiology and Chronic Health Evaluation Ⅱ score and the Glasgow Coma Scale score. Crit Care Med, 2000, 28(1): 120-124. doi: 10.1097/00003246-200001000-00020.
[24] CAO W, REN H, SONG B, et al. Red cell distribution width and Glasgow coma scale score as predictors of in-hospital mortality in maintenance hemodialysis patients diagnosed with spontaneous intracerebral hemorrhage. Medicine (Baltimore), 2022, 101(42): e31094. doi: 10.1097/MD.0000000000031094.
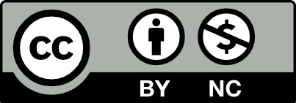
开放获取 本文遵循知识共享署名—非商业性使用4.0国际许可协议(CC BY-NC 4.0),允许第三方对本刊发表的论文自由共享(即在任何媒介以任何形式复制、发行原文)、演绎(即修改、转换或以原文为基础进行创作),必须给出适当的署名,提供指向本文许可协议的链接,同时标明是否对原文作了修改;不得将本文用于商业目的。CC BY-NC 4.0许可协议详情请访问 https://creativecommons.org/licenses/by-nc/4.0