Preoperative Evaluation of Cervical Lymph Node Metastasis in Patients With Hashimoto's Thyroiditis Combined With Thyroid Papillary Carcinoma Using Machine Learning and Radiomics-Based Features: A Preliminary Study
-
摘要:目的
利用机器学习(machine learning, ML)模型分析桥本甲状腺炎(Hashimoto thyroiditis, HT)合并甲状腺乳头状癌(papillary thyroid carcinoma, PTC)患者的甲状腺肿瘤的二维超声图像提取的影像组学和临床特征,探讨其术前无创识别该类患者颈部淋巴结转移(lymph node metastasis, LNM)的能力。
方法纳入HT合并PTC患者528例,以病理结果为金标准划分为存在颈部淋巴结转移组和不存在颈部淋巴结转移组,由3名医生独立勾画感兴趣区,提取感兴趣区的影像组学特征,以影像组学特征和影像组学特征结合临床特征2种模式构建随机森林(random forest, RF)、支持向量机(support vector machine, SVM)、LightGBM、K邻近算法(K-nearest neighbor, KNN)和XGBoost模型,在测试集上绘制受试者操作特征(receiver operating characteristic, ROC)曲线评价5种机器学习模型的2种模式的性能,并使用SHapley可加性解释(SHapley Additive exPlanations, SHAP)对模型进行可视化。
结果5种机器学习模型均具有较好的性能,ROC曲线下面积(area under curve, AUC)为0.798~0.921,其中LightGBM和XGBoost性能最佳,优于其他模型(P<0.05)。影像组学特征结合临床特征构建的机器学习模型优于仅使用影像组学特征构建的模型(P<0.05)。SHAP对性能最佳的模型可视化表明,前后径、上下径、original_shape_VoxelVolume、年龄、wavelet-LHL_firstorder_10Percentile和左右径对LightGBM的影响最显著;上下径、前后径、左右径、original_shape_VoxelVolume、original_firstorder_InterquartileRange和年龄对XGBoost的影响最显著。
结论基于影像组学和临床特征的机器学习模型能够准确地评估HT合并PTC患者颈部淋巴结状态。在5种机器学习模型中,LightGBM和XGBoost的评估性能最佳。
Abstract:ObjectiveTo analyze the radiomic and clinical features extracted from 2D ultrasound images of thyroid tumors in patients with Hashimoto's thyroiditis (HT) combined with papillary thyroid carcinoma (PTC) using machine learning (ML) models, and to explore the diagnostic performance of the method in making preoperative noninvasive identification of cervical lymph node metastasis (LNM).
MethodsA total of 528 patients with HT combined with PTC were enrolled and divided into two groups based on their pathological results of the presence or absence of LNM. The groups were subsequently designated the With LNM Group and the Without LNM Group. Three ultrasound doctors independently delineated the regions of interest and extracted radiomic features. Two modes, radiomic features and radiomics-clinical features, were used to construct random forest (RF), support vector machine (SVM), LightGBM, K-nearest neighbor (KNN), and XGBoost models. The performance of these five ML models in the two modes was evaluated by the receiver operating characteristic (ROC) curves on the test dataset, and SHapley Additive exPlanations (SHAP) was used for model visualization.
ResultsAll five ML models showed good performance, with area under the ROC curve (AUC) ranging from 0.798 to 0.921. LightGBM and XGBoost demonstrated the best performance, outperforming the other models (P<0.05). The ML models constructed with radiomics-clinical features performed better than those constructed using only radiomic features (P<0.05). The SHAP visualization of the best-performing models indicated that the anteroposterior diameter, superoinferior diameter, original_shape_VoxelVolume, age, wavelet-LHL_firstorder_10Percentile, and left-to-right diameter had the most significant effect on the LightGBM model. On the other hand, the superoinferior diameter, anteroposterior diameter, left-to-right diameter, original_shape_VoxelVolume, original_firstorder_InterquartileRange, and age had the most significant effect on the XGBoost model.
ConclusionML models based on radiomics and clinical features can accurately evaluate the cervical lymph node status in patients with HT combined with PTC. Among the 5 ML models, LightGBM and XGBoost demonstrate the best evaluation performance.
-
Keywords:
- Thyroid papillary carcinoma /
- Hashimoto thyroiditis /
- Machine learning /
- Radiomics
-
目前,甲状腺癌的发病率在中高收入国家中快速上升,已成为公共卫生中一个日益重要的问题[1-2],甲状腺乳头状癌(papillary thyroid carcinoma, PTC)的规范管理引起外科医生的广泛关注[3-4]。对于PTC管理,“少即是多”的概念已逐渐被外科医生接受[5-6]。桥本甲状腺炎(Hashimoto thyroiditis, HT)是一种对自身甲状腺组织产生抗体并导致甲状腺受损的自身免疫性疾病,且患者的颈部淋巴结常出现反应性增生[7-8]。而颈部淋巴结通常是PTC转移的首站[9]。随着HT和PTC的伴发增加,在术前区分HT合并PTC患者颈部淋巴结的良恶性对目前“少即是多”的PTC管理策略提出挑战[10]。最近研究[11-15]探究了HT合并PTC的危险因素,包括年龄、病灶数量、肿瘤直径大、甲状腺外扩张、颈部淋巴结肿大、肿瘤内部微钙化等,并构建相应的列线图,其测试集的受试者操作特征(receiver operating characteristic, ROC)曲线下面积(area under curve, AUC)仅为0.781~0.815。近年来,计算机硬件的进步促进了计算机辅助诊断系统的发展[14]。超声影像组学[16-18]能从甲状腺超声图像中提取肉眼无法识别的高通量特征,如纹理、边界和小波等特征,并结合机器学习模型以帮助临床诊断。本研究目的是开发一种机器学习模型,使用HT合并PTC患者的甲状腺结节的二维超声图像提取影像组学特征并结合临床特征来预测该患者是否存在颈部淋巴结转移(lymph node metastasis, LNM),以助力于HT合并PTC患者的临床管理。
1. 资料与方法
1.1 研究对象
研究纳入重庆医科大学附属第二医院2018年6月−2023年6月之间收治的HT合并PTC患者528例。纳入标准:①经手术证实为HT合并PTC;②年龄≥18岁;③在超声检查后30 d内进行手术;④临床数据完整。排除标准:①图像质量差;②超声检查结果在位置或大小上与病理检查结果不符;③超声图像上存在测量线;④术前接受过化疗、放射治疗或激素治疗等治疗的患者;⑤多灶性病变淋巴结病理结果对应不明确;⑥患其他恶性肿瘤病史。本研究获得重庆医科大学附属第二医院伦理委员会批准,批准号2024年研伦审第37号。
1.2 数据收集方法
收集患者的超声图像、临床特征及术前检查结果,包括年龄、性别、PTC术前二维超声图像、PTC术前超声检测前后径、左右径及上下径以及恶性颈部淋巴结的病理结果。
所有超声图像由GE Healthcare(LOGIQ E9, LOGIQ S7)、三星(RS80A)、迈瑞(Resona 7T)和飞利浦(EPIQ5、EPIQ7、IU22、IU Elite)超声仪采集,并从重庆医科大学附属第二医院的图片存档和通信系统工作站中提取图像。
最终共528例患者纳入研究。其中采用随机数法随机选取376例患者的978张PTC超声图像作为训练集,剩余的152例患者的198张超声图像作为测试集。所有符合纳入标准的患者都接受了手术治疗,并由两名病理科医生对标本进行病理诊断。
1.3 影像组学特征提取及筛选
1.3.1 影像组学特征提取及重复性估计
3名具有3~5年工作经验的超声科医生独立使用Labelme(v4.6.0)进行病变边界勾画。为了评估特征的稳定性,从训练集中随机选择40例患者,以计算特征组内相关系数(intraclass correlation coefficient, ICC)。
采用专门开源包(PyRadiomics,v3.1.0)[19]提取超声图像中的影像组学特征,提取二维形状、一阶特征、灰度共生矩阵(gray level co-occurrence matrix, GLCM)、灰度区域大小矩阵特征(gray level size zone matrix, GLSZM)、灰度行程矩阵特征(gray level run-length matrix, GLRLM)、邻域灰度差矩阵特征(neighbourhood gray-tone difference matrix, NGTDM)、灰度相关矩阵(gray level dependence matrix, GLDM)、小波变换、平方及梯度特征。可在官方文档(https://pyradiomics.readthedocs.io/en/latest/features.html)中找到所提取特征的公式和定义。
1.3.2 影像组学特征筛选
低方差(≤0.01)的非信息性特征被排除。类似地,基于成对相关矩阵丢弃高度相关(r≥0.75)的特征。使用两独立样本t检验筛选出P<0.05的特征。对训练集采用合成少数过采样技术(synthetic minority over-sampling technique, SMOTE)来平衡两种类型的数据。SMOTE通过对来自具有相同标签的原始群体的K-近邻的数据进行内插来创建少数群体的新样本(即合成患者)。重复这个过程,直到两个分类完全平衡。然后对数据进行标准化,最后,采用分层10次交叉验证递归特征消除(RFECV)和logistic回归(LBFGS求解器)来确定训练机器学习算法的最佳参数个数。
1.4 模型设计
本研究使用了随机森林(random forest, RF)、支持向量机(support vector machine, SVM)、LightGBM、K邻近算法(K-nearest neighbor, KNN)、XGBoost这5种机器学习算法[20-22]对影像组学和临床特征进行训练。采用optuna分别对每个机器学习模型的超参数进行调整,并对训练集使用五折交叉验证,选取准确率最高时所对应的参数为最佳参数构建最佳模型,并在测试集上测试最佳模型的性能。最后采用SHapley可加性解释(SHapley Additive exPlanations, SHAP) [23]对最佳性能的机器学习模型进行可视化。
1.5 统计学方法
为评估机器学习模型的性能,本研究使用约登指数(Youden index)确定最佳分类阈值,然后应用该阈值将模型输出概率分类为真阳性(true positives, TP)、假阳性(false positives, FP)、真阴性(true negatives, TN)和假阴性(false negatives, FN)。TP和TN表示正确分类的正负样本的数量,而FP和FN表示错误分类的正负样本的数量。在这项研究中,阳性样本指的是HT合并PTC且存在淋巴结转移的患者。本研究还绘制ROC曲线,并计算AUC,以评估模型在测试集上的整体性能。为确定是否不同模型之间的LNM评估有显著差异,使用DeLong检验。此外,还使用F1值、敏感性(sensitivity)、准确率(accuracy)、特异性(specificity)、阳性预测值(positive predictive value, PPV)和阴性预测值(negative predictive value, NPV)等量化指标来进一步评估模型的性能。这些评价指标的定义如下:
$$ {F}_{1}=2\times ({\mathrm{precision}}\times {\mathrm{recall}})/({\mathrm{precision}}+{\mathrm{recall}}) $$ (1) $$ {\mathrm{accuracy=(TP+TN)/(TP+TN+FP+FN)}} $$ (2) $$ {\mathrm{specificity=TN/(TN+FP)}} $$ (3) $$ {\mathrm{sensitivity=TP/(TP+FN)}} $$ (4) $$ {\mathrm{PPV=TP/(TP+FP)}} $$ (5) $$ {\mathrm{NPV=TN/(TN+FN)}} $$ (6) 使用威尔逊方法(Wilson's method)计算95%的置信区间(confidence interval, CI),并认为双尾P值小于0.05为差异有统计学意义。使用MedCalc(v22.001)和SPSS(v25.0)进行统计分析。
2. 结果
2.1 基本资料
本研究纳入HT合并PTC患者528例的
1176 张超声图像,随机选取376例患者的978张PTC超声图像作为训练集,152例患者的198张超声图像作为测试集。HT合并PTC存在颈部淋巴结转移的患者与不存在颈部淋巴结转移的患者的临床资料如表1所示。两组间年龄、性别及PTC的左右径、前后径及上下径的差异均有统计学意义(P<0.05)。表 1 患者基本资料Table 1. Baseline data of the patients enrolledCharacteristic With LNM
(n=189)Without LNM
(n=339)P Sex/case <0.001 Female 168 329 Male 21 10 Age/yr. 39.93±11.93 44.10±11.92 0.0013 Transverse diameter/case <0.001 ≤1 cm 107 297 >1 cm 82 42 Anteroposterior diameter/case <0.001 ≤1 cm 124 307 >1 cm 65 32 Superoinferior diameter/case <0.001 ≤1 cm 90 279 >1 cm 99 60 LNM: lymph node metastasis. 2.2 机器学习模型构建
2.2.1 影像组学特征筛选
一共提取
1331 个特征,在这些特征中,排除459个低方差特征和798个高度相关的特征,然后,通过两独立样本t检验排除了28个特征。在采用SMOTE对训练集进行分类平衡后,RFECV从剩余的46个特征中识别出16个特征的子集,包括“original_shape_VoxelVolume”“original_firstorder_90Percentile”“original_firstorder_InterquartileRange”“original_glcm_Contrast”“wavelet-LLH_firstorder_Entropy”“wavelet-LLH_firstorder_Skewness”“wavelet-LLH_glcm_JointEntropy”“wavelet-LLH_gldm_DependenceVariance”“wavelet-LHL_firstorder_10Percentile”“wavelet-LHL_glcm_ClusterProminence”“wavelet-LHH_gldm_SmallDependenceHighGrayLevelEmphasis”“wavelet-HLL_firstorder_Entropy”“wavelet-HLL_firstorder_Skewness”“wavelet-HLL_glcm_Autocorrelation”“wavelet-HLL_gldm_DependenceEntropy”“wavelet-HLL_gldm_SmallDependenceHighGrayLevelEmphasis”。图1展示了所提取的16个影像组学特征的皮尔逊相关系数热力图。2.2.2 影像组学特征构建机器学习模型性能
在训练集中,RF、SVM、LightGBM、KNN和XGBoost的准确度分别为0.748、0.835、0.816、0.814和0.838。在测试集中,RF、SVM、LightGBM、KNN和XGBoost的诊断HT合并PTC的AUC分别为0.747 (95%CI:0.678~0.817)、0.682 (95%CI:0.605~0.758)、0.711 (95%CI:0.635~0.787)、0.700 (95%CI:0.624~0.775)和0.727 (95%CI:0.654~0.800)(图2A)。经DeLong检验,5种模型性能差异无统计学意义(P>0.05)。此外,还计算F1值、敏感性、准确率、特异性、PPV和NPV进一步评估模型的性能(表2)。
表 2 影像组学特征构建的5种机器学习模型性能Table 2. Performance of five machine learning models constructed with radiomics featuresModel AUC (95% CI) Sensitivity (95% CI) Specificity (95% CI) PPV (95% CI) NPV (95% CI) Accuracy (95% CI) F1 RF 0.747 (0.678-0.817) 0.723 (0.609-0.820) 0.590 (0.498-0.678) 0.524 (0.460-0.587) 0.774 (0.698-0.835) 0.641 (0.570-0.708) 0.608 SVM 0.682 (0.605-0.758) 0.645 (0.527-0.751) 0.639 (0.547-0.724) 0.527 (0.455-0.598) 0.743 (0.675-0.801) 0.641 (0.570-0.708) 0.580 LightGBM 0.711 (0.635-0.787) 0.684 (0.567-0.786) 0.566 (0.473-0.655) 0.495 (0.432-0.558) 0.742 (0.666-0.806) 0.611 (0.539-0.679) 0.575 KNN 0.700 (0.624-0.775) 0.855 (0.756-0.925) 0.426 (0.337-0.519) 0.481 (0.437-0.526) 0.825 (0.725-0.894) 0.591 (0.519-0.660) 0.616 XGBoost 0.727 (0.654-0.800) 0.724 (0.601-0.820) 0.582 (0.489-0.671) 0.519 (0.456-0.581) 0.636 (0.565-0.703) 0.772 (0.695-0.834) 0.604 AUC: area under curve; CI: confidence interval; PPV: positive predictive value; NPV: negative predictive value. 2.2.3 影像组学特征结合临床特征构建机器学习模型
采用与前文相同的影像组学特征筛选方法筛选出16个特征后加上了包括年龄,甲状腺结节左右径、前后径、上下径在内的临床特征,形成20个相关特征进行模型构建。在训练集上RF、SVM、LightGBM、KNN和XGBoost的准确度分别为0.841、0.899、0.934、0.892和0.934。在测试集上,通过影像组学特征结合临床特征构建的RF、SVM、LightGBM、KNN和XGBoost的AUC分别为0.884 (95%CI:0.839~0.930)、0.798 (95%CI:0.734~0.863)、0.921 (95%CI:0.885~0.957)、0.813 (95%CI:0.747~0.878)和0.910 (95%CI:0.871~0.950) (图2B)。此外,经DeLong检验,LightGBM和XGBoost的AUC值优于其他机器学习模型(P<0.05)。影像组学特征结合临床特征构建的机器学习模型的F1值、敏感性、准确率、特异性、PPV和NPV值如表3 所示。
表 3 影像组学特征结合临床特征构建的5种机器学习模型性能Table 3. Performance of five machine learning models for radiomics-clinical featuresModel AUC (95% CI) Sensitivity (95% CI) Specificity (95% CI) PPV (95% CI) NPV (95% CI) Accuracy (95% CI) F1 RF 0.884 (0.839-0.930) 0.605 (0.487-0.716) 0.951 (0.896-0.982) 0.885 (0.775-0.945) 0.795 (0.745-0.837) 0.818 (0.757-0.869) 0.719 SVM 0.798 (0.734-0.863) 0.553 (0.434-0.667) 0.893 (0.825-0.942) 0.764 (0.650-0.849) 0.762 (0.713-0.806) 0.763 (0.697-0.820) 0.641 LightGBM 0.921 (0.885-0.957) 0.697 (0.581-0.798) 0.902 (0.834-0.948) 0.815 (0.717-0.885) 0.827 (0.772-0.871) 0.823 (0.763-0.874) 0.752 KNN 0.813 (0.747-0.878) 0.737 (0.623-0.831) 0.795 (0.713-0.863) 0.691 (0.606-0.765) 0.829 (0.767-0.877) 0.773 (0.708-0.829) 0.713 XGBoost 0.910 (0.871-0.950) 0.645 (0.527-0.751) 0.918 (0.854-0.960) 0.831 (0.726-0.901) 0.806 (0.753-0.849) 0.813 (0.752-0.865) 0.726 AUC: area under curve; CI: confidence interval; PPV: positive predictive value; NPV: negative predictive value. 此外,采用DeLong 检验对比评估影像组学模型与影像组学结合临床特征模型的性能,影像组学特征结合临床特征在5种机器学习模型中,AUC均优于单独的影像组学特征(P<0.05)(表4)。
表 4 DeLong检验比较影像组学模型和影像组学结合临床特征模型性能Table 4. Performance comparison of the radiomics models and the radiomics-clinical models using the DeLong's TestModel Radiomics models
(AUC [95% CI])Radiomics-clinical models
(AUC [95% CI])P RF 0.747 (0.678-0.817) 0.884 (0.839-0.930) < 0.0001 SVM 0.682 (0.605-0.758) 0.798 (0.734-0.863) 0.0067 LightGBM 0.711 (0.635-0.787) 0.921 (0.885-0.957) < 0.0001 KNN 0.700 (0.624-0.775) 0.813 (0.747-0.878) 0.0070 XGBoost 0.727 (0.654-0.800) 0.910 (0.871-0.950) < 0.0001 2.3 SHAP可视化最佳机器学习模型
为使机器学习模型更直观和具有可解释性,使用SHAP分析可视化性能最佳的LightGBM和XGBoost模型。SHAP图中展示特征的重要性和解释对预测结果的影响。图3A为条形图,该图展现特征的重要性和影响,特征按照其对预测结果的整体影响进行排序;SHAP蜂群图(图3B)展现每个特征的数据点在y轴上分布,水平位置表示对预测结果的影响。蜂群图上的不同颜色表示每个特征对预测结果的正面或负面影响。较红的色调表示该特征的SHAP值较高,而较蓝的颜色表示SHAP值较低。结果表明,在LightGBM中,前后径、上下径、original_shape_VoxelVolume、年龄、wavelet-LHL_firstorder_10Percentile和左右径这6个特征对模型的影响最显著;上下径、前后径、左右径、original_shape_VoxelVolume、original_firstorder_InterquartileRange和年龄6个特征对XGBoost模型的影响最显著。
图 3 LightGBM和XGBoost模型的SHAP图Figure 3. SHAP plots for LightGBM and XGBoost modelsA, The bar charts of the SHAP summary plots for the LightGBM model displays the impact of each feature on the LightGBM model. Class 0: without lymph node metastasis (LNM); Class 1: with LNM. B, The scatter plot in the SHAP summary plots for the XGBoost model visualizes the relationship between feature values and predicted outcomes using colors, including both positive and negative predictive effects.3. 讨论
本研究基于RF、SVM、LightGBM、KNN和XGBoost模型,将HT合并PTC患者的甲状腺肿瘤超声图像提取的影像组学特征分别输入到5种机器学习模型中进行分析,并对比仅基于影像组学特征和影像组学结合临床特征所训练的模型性能,此外,还通过SHAP对模型进行可解释性分析。本研究结果表明,机器学习模型能够很好地学习影像组学和临床特征并对患者进行术前无创评估颈部淋巴结状态,且与单独的影像组学模型相比,影像组学结合临床特征模型能更好地对颈部淋巴结状态做出评估。此外,SHAP对模型进行进一步的可视化和解释,减少机器学习模型的“黑匣子”属性。本研究表明基于影像组学和临床特征结合机器学习模型评价HT合并PTC患者颈部淋巴结转移的可行性,为早期无创评价淋巴结状态提供新思路。
随着超声技术的发展,甲状腺肿瘤的检出率近年来急剧上升[1-2]。然而,对其过度诊断和治疗引起临床广泛的关注。大量研究[24-28]致力于探索对甲状腺癌,特别是PTC的最佳管理方式。HT作为一种常见的自身免疫性疾病常引起颈部淋巴结肿大,HT合并PTC的患者颈部淋巴结肿大原因对外科医生和超声科医生提出了挑战。因此,无创评估HT合并PTC患者颈部淋巴结状态对PTC的临床管理至关重要。
目前已有探究HT合并PTC患者颈部淋巴结状态评估的相关研究[9-13, 28-29]。MIN等[13] 采用单因素和多因素logistic回归分析,确定高水平血清甲状腺球蛋白抗体(TgAb)、肿瘤部位较低、淋巴结边缘不规则、淋巴结内微钙化是PTC患者淋巴结转移的危险因素。然而与上述研究不同的是,ZHAO等[12]研究表明,年龄较小、体质量指数正常、BRAF V600E突变、最大直径较大、左叶肿瘤、纵横比>1、包膜侵犯和钙化是PTC患者发生中央淋巴结转移的显著危险因素。李惠等[29]发现年龄较小、结节性高回声、肿瘤大直径、肿瘤多灶性、甲状腺外扩张、颈部淋巴结肿大、癌胚抗原阳性是发生淋巴结转移的独立预测因素。不同研究对HT合并PTC患者颈部淋巴结转移危险因素的评估结果存在不一致的问题[12-13, 30]。此外,JIN等[11]使用患者的临床资料和超声影像组学特征建立诺模图以预测颈部淋巴结转移,但最佳模型在测试集上的AUC仅为0.808。而本研究提出的机器学习模型获得了较高的AUC,最高可达0.921。
本研究仍存在一些不足。首先,由于本研究的样本量有限,存在样本不平衡的问题,因此本研究主要将AUC作为模型的评价指标,在之后的研究中应该扩大样本量。其次,本研究为回顾性研究且只纳入了单张超声图像,需要进行前瞻性研究并纳入更多的临床参数和动态超声视频。最后,本研究为单中心研究,虽然已随机划分了测试集,但仍需要外部验证对模型性能进行进一步地评估。
综上所述,影像组学结合临床特征构建的机器学习模型可较准确地无创评估颈部淋巴结状态,有利于HT合并PTC患者的临床管理,具有良好的可行性和临床应用价值。
* * *
作者贡献声明 付汝倩负责论文构思、正式分析和初稿写作,邓诗负责数据审编和初稿写作,胡宇婷负责研究调查,罗朋和杨浩负责验证,滕花负责初稿写作和审读与编辑写作,曾德智负责可视化,任建丽负责经费获取、研究项目管理和审读与编辑写作。所有作者已经同意将文章提交给本刊,且对将要发表的版本进行最终定稿,并同意对工作的所有方面负责。
Author Contribution FU Ruqian is responsible for conceptualization, formal analysis, and writing--original draft. DENG Shi is responsible for data curation and writing--original draft. HU Yuting is responsible for investigation. LUO Peng and YANG Hao are responsible fo validation. TENG Hua is responsible for writing--original draft and writing--review and editing. ZENG Dezhi is responsible for visualization. REN Jianli is responsible for funding acquisition, project administration, and writing--review and editing. All authors consented to the submission of the article to the Journal. All authors approved the final version to be published and agreed to take responsibility for all aspects of the work.
利益冲突 所有作者均声明不存在利益冲突
Declaration of Conflicting Interests All authors declare no competing interests.
-
图 3 LightGBM和XGBoost模型的SHAP图
Figure 3. SHAP plots for LightGBM and XGBoost models
A, The bar charts of the SHAP summary plots for the LightGBM model displays the impact of each feature on the LightGBM model. Class 0: without lymph node metastasis (LNM); Class 1: with LNM. B, The scatter plot in the SHAP summary plots for the XGBoost model visualizes the relationship between feature values and predicted outcomes using colors, including both positive and negative predictive effects.
表 1 患者基本资料
Table 1 Baseline data of the patients enrolled
Characteristic With LNM
(n=189)Without LNM
(n=339)P Sex/case <0.001 Female 168 329 Male 21 10 Age/yr. 39.93±11.93 44.10±11.92 0.0013 Transverse diameter/case <0.001 ≤1 cm 107 297 >1 cm 82 42 Anteroposterior diameter/case <0.001 ≤1 cm 124 307 >1 cm 65 32 Superoinferior diameter/case <0.001 ≤1 cm 90 279 >1 cm 99 60 LNM: lymph node metastasis. 表 2 影像组学特征构建的5种机器学习模型性能
Table 2 Performance of five machine learning models constructed with radiomics features
Model AUC (95% CI) Sensitivity (95% CI) Specificity (95% CI) PPV (95% CI) NPV (95% CI) Accuracy (95% CI) F1 RF 0.747 (0.678-0.817) 0.723 (0.609-0.820) 0.590 (0.498-0.678) 0.524 (0.460-0.587) 0.774 (0.698-0.835) 0.641 (0.570-0.708) 0.608 SVM 0.682 (0.605-0.758) 0.645 (0.527-0.751) 0.639 (0.547-0.724) 0.527 (0.455-0.598) 0.743 (0.675-0.801) 0.641 (0.570-0.708) 0.580 LightGBM 0.711 (0.635-0.787) 0.684 (0.567-0.786) 0.566 (0.473-0.655) 0.495 (0.432-0.558) 0.742 (0.666-0.806) 0.611 (0.539-0.679) 0.575 KNN 0.700 (0.624-0.775) 0.855 (0.756-0.925) 0.426 (0.337-0.519) 0.481 (0.437-0.526) 0.825 (0.725-0.894) 0.591 (0.519-0.660) 0.616 XGBoost 0.727 (0.654-0.800) 0.724 (0.601-0.820) 0.582 (0.489-0.671) 0.519 (0.456-0.581) 0.636 (0.565-0.703) 0.772 (0.695-0.834) 0.604 AUC: area under curve; CI: confidence interval; PPV: positive predictive value; NPV: negative predictive value. 表 3 影像组学特征结合临床特征构建的5种机器学习模型性能
Table 3 Performance of five machine learning models for radiomics-clinical features
Model AUC (95% CI) Sensitivity (95% CI) Specificity (95% CI) PPV (95% CI) NPV (95% CI) Accuracy (95% CI) F1 RF 0.884 (0.839-0.930) 0.605 (0.487-0.716) 0.951 (0.896-0.982) 0.885 (0.775-0.945) 0.795 (0.745-0.837) 0.818 (0.757-0.869) 0.719 SVM 0.798 (0.734-0.863) 0.553 (0.434-0.667) 0.893 (0.825-0.942) 0.764 (0.650-0.849) 0.762 (0.713-0.806) 0.763 (0.697-0.820) 0.641 LightGBM 0.921 (0.885-0.957) 0.697 (0.581-0.798) 0.902 (0.834-0.948) 0.815 (0.717-0.885) 0.827 (0.772-0.871) 0.823 (0.763-0.874) 0.752 KNN 0.813 (0.747-0.878) 0.737 (0.623-0.831) 0.795 (0.713-0.863) 0.691 (0.606-0.765) 0.829 (0.767-0.877) 0.773 (0.708-0.829) 0.713 XGBoost 0.910 (0.871-0.950) 0.645 (0.527-0.751) 0.918 (0.854-0.960) 0.831 (0.726-0.901) 0.806 (0.753-0.849) 0.813 (0.752-0.865) 0.726 AUC: area under curve; CI: confidence interval; PPV: positive predictive value; NPV: negative predictive value. 表 4 DeLong检验比较影像组学模型和影像组学结合临床特征模型性能
Table 4 Performance comparison of the radiomics models and the radiomics-clinical models using the DeLong's Test
Model Radiomics models
(AUC [95% CI])Radiomics-clinical models
(AUC [95% CI])P RF 0.747 (0.678-0.817) 0.884 (0.839-0.930) < 0.0001 SVM 0.682 (0.605-0.758) 0.798 (0.734-0.863) 0.0067 LightGBM 0.711 (0.635-0.787) 0.921 (0.885-0.957) < 0.0001 KNN 0.700 (0.624-0.775) 0.813 (0.747-0.878) 0.0070 XGBoost 0.727 (0.654-0.800) 0.910 (0.871-0.950) < 0.0001 -
[1] LORTET-TIEULENT J, FRANCESCHI S, DAL MASO L, et al. Thyroid cancer "epidemic" also occurs in low- and middle-income countries. Int J Cancer, 2019, 144(9): 2082–2087. doi: 10.1002/ijc.31884.
[2] MIRANDA-FILHO A, LORTET-TIEULENT J, BRAY F, et al. Thyroid cancer incidence trends by histology in 25 countries: a population-based study. Lancet Diabetes Endocrinol, 2021, 9(4): 225–234. doi: 10.1016/S2213-8587(21)00027-9.
[3] HADDAD R I, BISCHOFF L, BALL D, et al. Thyroid carcinoma, version 2. 2022, NCCN clinical practice guidelines in oncology. J Natl Compr Canc Netw, 2022, 20(8): 925–951. doi: 10.6004/jnccn.2022.0040.
[4] BOUCAI L, ZAFEREO M, CABANILLAS M E. Thyroid cancer: a review. JAMA, 2024, 331(5): 425–435. doi: 10.1001/jama.2023.26348.
[5] CHO S J, SUH C H, BAEK J H, et al. Active surveillance for small papillary thyroid cancer: a systematic review and meta-analysis. Thyroid, 2019, 29(10): 1399–1408. doi: 10.1089/thy.2019.0159.
[6] SUGITANI I, ITO Y, TAKEUCHI D, et al. Indications and strategy for active surveillance of adult low-risk papillary thyroid microcarcinoma: Consensus Statements from the Japan Association of Endocrine Surgery Task Force on Management for Papillary Thyroid Microcarcinoma. Thyroid, 2021, 31(2): 183–192. doi: 10.1089/thy.2020.0330.
[7] XU S, HUANG H, QIAN J, et al. Prevalence of Hashimoto thyroiditis in adults with papillary thyroid cancer and its association with cancer recurrence and outcomes. JAMA Netw Open, 2021, 4(7): e2118526. doi: 10.1001/jamanetworkopen.2021.18526.
[8] 李云华, 杜联芳, 常才. 超声对桥本甲状腺炎合并良恶性结节的鉴别诊断价值. 中华超声影像学杂志, 2019, 28(12): 1093–1097. doi: 10.3760/cma.j.issn.1004-4477.2019.12.017. LI Y H, DU L F, CHANG C. Value of ultrasonography in the differential diagnosis of benign and malignant thyroid nodules with Hashimoto's thyroiditis. Chin J Ultrason, 2019, 28(12): 1093–1097. doi: 10.3760/cma.j.issn.1004-4477.2019.12.017.
[9] HENG Y, YANG Z, ZHOU L, et al. Risk stratification for lateral involvement in papillary thyroid carcinoma patients with central lymph node metastasis. Endocrine, 2020, 68(2): 320–328. doi: 10.1007/s12020-020-02194-8.
[10] 车勇军, 连蕾, 侯钰, 等. 桥本氏甲状腺炎合并甲状腺乳头状癌患者的临床病理特征及其与BRAF基因突变的相关性研究. 中国耳鼻咽喉头颈外科, 2023, 30(2): 69–73. doi: 10.16066/j.1672-7002.2023.02.001. CHEN Y J, LIAN L, HOU Y, et al. The clinicopathology and characteristic expression analysis of HT combined with PTC and its correlation with BRAF gene mutation. Chin Arch Otolaryngol Head Neck Surg, 2023, 30(2): 69–73. doi: 10.16066/j.1672-7002.2023.02.001.
[11] JIN P, CHEN J, DONG Y, et al. Ultrasound-based radiomics nomogram combined with clinical features for the prediction of central lymph node metastasis in papillary thyroid carcinoma patients with Hashimoto's thyroiditis. Front Endocrinol (Lausanne), 2022, 13: 993564. doi: 10.3389/fendo.2022.993564.
[12] ZHAO W, HE L, ZHU J, et al. A nomogram model based on the preoperative clinical characteristics of papillary thyroid carcinoma with Hashimoto's thyroiditis to predict central lymph node metastasis. Clin Endocrinol (Oxf), 2021, 94(2): 310–321. doi: 10.1111/cen.14302.
[13] MIN Y, HUANG Y, WEI M, et al. Preoperatively predicting the central lymph node metastasis for papillary thyroid cancer patients with Hashimoto's thyroiditis. Front Endocrinol (Lausanne), 2021, 12: 713475. doi: 10.3389/fendo.2021.713475.
[14] 冯嘉伟, 叶晶, 胡俊, 等. 基于临床和超声特征预测甲状腺微小乳头状癌中央淋巴结转移的Nomogram. 重庆医科大学学报, 2022, 47(11): 1282–1288. doi: 10.13406/j.cnki.cyxb.003129. FENG J W, YE J, HU J, et al. A nomogram based on clinical and ultrasound characteristics to predict central lymph node metastases of papillary thyroid microcarcinoma. J Chongqing Med Univ, 2022, 47(11): 1282–1288. doi: 10.13406/j.cnki.cyxb.003129.
[15] HA E J, LEE J H, LEE D H, et al. Development of a machine learning-based fine-grained risk stratification system for thyroid nodules using predefined clinicoradiological features. Eur Radiol, 2023, 33(5): 3211–3221. doi: 10.1007/s00330-022-09376-0.
[16] ROMEO V, CUOCOLO R, APOLITO R, et al. Clinical value of radiomics and machine learning in breast ultrasound: a multicenter study for differential diagnosis of benign and malignant lesions. Eur Radiol, 2021, 31(12): 9511–9519. doi: 10.1007/s00330-021-08009-2.
[17] LI M D, CHENG M Q, CHEN L D, et al. Reproducibility of radiomics features from ultrasound images: influence of image acquisition and processing. Eur Radiol, 2022, 32(9): 5843–5851. doi: 10.1007/s00330-022-08662-1.
[18] DURON L, SAVATOVSKY J, FOURNIER L, et al. Can we use radiomics in ultrasound imaging? Impact of preprocessing on feature repeatability. Diagn Interv Imaging, 2021, 102(11): 659–667. doi: 10.1016/j.diii.2021.10.004.
[19] Van TIMMEREN J E, CESTER D, TANADINI-LANG S, et al. Radiomics in medical imaging-"how-to" guide and critical reflection. Insights Imaging, 2020, 11(1): 91. doi: 10.1186/s13244-020-00887-2.
[20] TIAN L, ZHANG D, BAO S, et al. Radiomics-based machine-learning method for prediction of distant metastasis from soft-tissue sarcomas. Clin Radiol, 2021, 76(2): 158.e19–158.e25. doi: 10.1016/j.crad.2020.08.038.
[21] MAO B, MA J, DUAN S, et al. Preoperative classification of primary and metastatic liver cancer via machine learning-based ultrasound radiomics. Eur Radiol, 2021, 31(7): 4576–4586. doi: 10.1007/s00330-020-07562-6.
[22] MISHRA A K, ROY P, BANDYOPADHYAY S, et al. Breast ultrasound tumour classification: a machine learning--radiomics based approach. Expert Systems, 2021, 38(7): e12713. doi: 10.1111/exsy.12713.
[23] LI J, XIA F, WANG X, et al. Multiclassifier radiomics analysis of ultrasound for prediction of extrathyroidal extension in papillary thyroid carcinoma in children. Int J Med Sci, 2023, 20(2): 278–286. doi: 10.7150/ijms.79758.
[24] FU R, YANG H, ZENG D, et al. PTC-MAS: a deep learning-based preoperative automatic assessment of lymph node metastasis in primary thyroid cancer. Diagnostics (Basel), 2023, 13(10): 1723. doi: 10.3390/diagnostics13101723.
[25] PENG S, LIU Y, LV W, et al. Deep learning-based artificial intelligence model to assist thyroid nodule diagnosis and management: a multicentre diagnostic study. Lancet Digit Health, 2021, 3(4): e250–e259. doi: 10.1016/s2589-7500(21)00041-8.
[26] BUDA M, WILDMAN-TOBRINER B, HOANG J K, et al. Management of thyroid nodules seen on US images: deep learning may match performance of radiologists. Radiology, 2019, 292(3): 695–701. doi: 10.1148/radiol.2019181343.
[27] LI X, ZHANG S, ZHANG Q, et al. Diagnosis of thyroid cancer using deep convolutional neural network models applied to sonographic images: a retrospective, multicohort, diagnostic study. Lancet Oncol, 2019, 20(2): 193–201. doi: 10.1016/s1470-2045(18)30762-9.
[28] QIAN X, PEI J, ZHENG H, et al. Prospective assessment of breast cancer risk from multimodal multiview ultrasound images via clinically applicable deep learning. Nat Biomed Eng, 2021, 5(6): 522–532. doi: 10.1038/s41551-021-00711-2.
[29] 李惠, 黄玉婷, 娄鹏威, 等. 甲状腺乳头状癌伴桥本甲状腺炎患者中央区淋巴结转移预测模型的构建与验证. 现代肿瘤医学, 2023, 31(12): 2239–2247. doi: 10.3969/j.issn.1672-4992.2023.12.012. LI H, HUANG Y T, LOU P W, et al. Establishment and validation of a risk-predictive model for central lymph node metasta-sis in papillary thyroid carcinoma with Hashimotos thyroiditis. J Mod Oncol, 2023, 31(12): 2239–2247. doi: 10.3969/j.issn.1672-4992.2023.12.012.
[30] 丁金旺, 潘钢, 项洋锋, 等. 甲状腺乳头状癌合并桥本甲状腺炎患侧中央区淋巴结转移的临床危险因素分析. 浙江医学, 2021, 43(15): 1643–1646. doi: 10.12056/j.issn.1006-2785.2021.43.15.2020-1500. DING J W, PAN G, XIANG Y F, et al. Risk factors of central lymph node metastasis in papillary thyroid cancer complicated with Hashimoto's thyroiditis. Zhejiang Med J, 2021, 43(15): 1643–1646. doi: 10.12056/j.issn.1006-2785.2021.43.15.2020-1500.
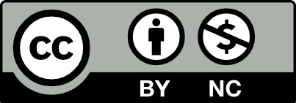
开放获取 本文遵循知识共享署名—非商业性使用4.0国际许可协议(CC BY-NC 4.0),允许第三方对本刊发表的论文自由共享(即在任何媒介以任何形式复制、发行原文)、演绎(即修改、转换或以原文为基础进行创作),必须给出适当的署名,提供指向本文许可协议的链接,同时标明是否对原文作了修改;不得将本文用于商业目的。CC BY-NC 4.0许可协议详情请访问 https://creativecommons.org/licenses/by-nc/4.0