Dynamic Prediction of Recidivism in Violence in Community-Based Schizophrenia Spectrum Disorder Patients: A Joint Model
-
摘要:目的
采用联合建模的策略,建立一种能预测社区精神分裂症谱系障碍患者(简称精分患者)暴力再犯的模型。
方法基于中国西南某地2017年1月–2018年6月重性精神疾病基本数据,选取基线存在暴力行为的
4565 名社区成年精分患者作为研究对象。采用生长混合模型识别服药依从性和社会功能模式,进一步采用零膨胀负二项回归模型拟合联合模型,并与传统静态模型进行比较,最后采用10折训练-测试分割交叉验证框架评价模型拟合和预测效果。结果157位(3.44%)患者发生暴力再犯。服药依从性和社会功能均拟合出4种模式。计数模型中,年龄、婚姻情况、教育水平、经济水平、历史暴力类型和服药依从性模式是暴力再犯发生次数的预测因素(P<0.05)。零膨胀模型中,年龄、药物不良反应、历史暴力类型、服药依从性模式和社会功能模式是暴力再犯是否发生的预测因素(P<0.05)。联合模型训练集赤池信息准则(Akaike information criterion, AIC)平均值为776.5±9.4、测试集均方根误差(root mean squared error, RMSE)平均值为0.168±0.013、平均绝对误差(mean absolute error, MAE)平均值为0.131±0.018,均小于传统静态模型。
结论联合建模是一种有效的动态变量识别与处理统计学策略,预测性能优于传统静态模型,可为推动综合干预体系建设提供全新思路。
-
关键词:
- 精神分裂症 /
- 暴力再犯 /
- 生长混合模型 /
- 零膨胀负二项回归模型
Abstract:ObjectiveTo construct a model for predicting recidivism in violence in community-based schizophrenia spectrum disorder patients (SSDP) by adopting a joint modeling method.
MethodsBased on the basic data on severe mental illness in Southwest China between January 2017 and June 2018,
4565 community-based SSDP with baseline violent behaviors were selected as the research subjects. We used a growth mixture model (GMM) to identify patterns of medication adherence and social functioning. We then fitted the joint model using a zero-inflated negative binomial regression model and compared it with traditional static models. Finally, we used a 10-fold training-test cross validation framework to evaluate the models’ fitting and predictive performance.ResultsA total of 157 patients (3.44%) experienced recidivism in violence. Medication compliance and social functioning were fitted into four patterns. In the counting model, age, marital status, educational attainment, economic status, historical types of violence, and medication compliance patterns were predictive factors for the frequency of recidivism of violence (P<0.05). In the zero-inflated model, age, adverse drug reactions, historical types of violence, medication compliance patterns, and social functioning patterns were predictive factors for the recidivism in violence (P<0.05). For the joint model, the average value of Akaike information criterion (AIC) for the train set was 776.5±9.4, the average value of root mean squared error (RMSE) for the testing set was 0.168±0.013, and the average value of mean absolute error (MAE) for the testing set was 0.131±0.018, which were all lower than those of the traditional static models.
ConclusionJoint modeling is an effective statistical strategy for identifying and processing dynamic variables, exhibiting better predictive performance than that of the traditional static models. It can provide new ideas for promoting the construction of comprehensive intervention systems.
-
精神分裂症谱系障碍患者(以下简称精分患者)因其对自身行为以及周围环境的控制和辨认能力下降甚至丧失被认为是暴力行为的重点人群[1-2]。暴力史是精分患者暴力行为最显著的预测因素之一[3-4]。重复暴力给司法和公共卫生系统带来了双重负担[5],如何有效预测暴力再犯值得深入研究,但国内相关研究起步较晚[6]。近年,多项研究指出社区患者与住院患者在暴力再犯的预测因素上存在差异[7-9],且现有关于社区患者暴力再犯相关的研究均集中在司法系统下的社区监禁情景[10],对于数量众多的一般社区患者不具有参考意义,因此社区患者暴力再犯预测仍然是基层公共卫生亟须解决的重点问题。
一般认为,精分患者暴力再犯主要与治疗质量有关[11],即治疗和康复能预防暴力再犯,因此,对具有可干预性的动态变量如自知力、服药依从性、社会功能等的研究不仅具有重要的预测意义[12],也能推动建设以治疗和康复为主的基层公共卫生综合性干预体系。然而,现有关于精分患者暴力再犯的研究仅局限于提出动态变量的概念[13],设计和分析层面依然使用横断面研究方式识别与处理动态变量[14],因此可能错误地估计了动态变量的效应。此外,大多数现有研究定义暴力再犯结局为二分类变量[8, 12, 14],忽视了重复发生的暴力再犯计数数据,因而可能导致统计效能降低。考虑到以上局限性,本研究拟采用一种联合建模策略对社区精分患者暴力再犯进行预测,采用生长混合模型(growth mixture modeling, GMM)识别动态变量潜在类别,并在此基础上通过零膨胀负二项回归模型(zero-inflated negative binomial regression model, ZINBRM)建立暴力再犯预测模型,为识别和处理动态变量提供一种新思路,同时也为社区建设个体化综合性干预体系提供实证与方法学参考依据。
1. 资料与方法
1.1 研究对象
研究对象来源于中国西南某地重性精神疾病基本数据收集分析系统,该系统由专业社区精神卫生工作人员于2006年1月–2018年6月收集和记录了基层卫生服务中心辖区内重性精神疾病患者的基本信息与随访信息,视患者病情稳定性至少每3个月收集一次随访信息。本研究收集了2017年1月–2018年6月的数据。研究对象的纳入标准为:①由专业精神科医师经《疾病和相关健康问题的国际统计分类(第十版)》(International Statistical Classification of Diseases and Related Health Problems, ICD-10)诊断为精神分裂症谱系障碍(ICD-10/F20-F29);②年龄≥18岁;③2017年1月前至少有过1次暴力行为。研究对象的排除标准是:①随访期间持续住院;②合并其他严重躯体疾病;③服药依从性、社会功能随访记录完全缺失;④结局随访记录完全缺失;⑤随访期间因非死亡原因失访。
4565 名社区成年精分患者符合本研究纳入标准。所有研究对象均由本人或法定监护人签署了同意参与数据收集与分析的纸质知情同意文件,研究方案与知情同意文件已经研究所属单位与精神卫生中心设计人类参与者的机构审查委员会批准。
1.2 研究变量
本研究主要结局指标为暴力再犯,指基线存在既往暴力的研究对象在随访期间再次出现以下一种或几种情况的次数:①轻度滋事:指公安机关出警但患者行为并未达到触犯《中华人民共和国治安管理处罚法》的标准;②肇事:患者行为触犯《中华人民共和国治安管理处罚法》;③肇祸:患者行为触犯《中华人民共和国刑法》;④自我伤害:无自杀意图的情况下患者对自身的蓄意伤害;⑤自杀未遂:具有自杀意图的自我伤害;⑥自杀。
其余变量:在基线时收集性别、民族、就业情况、婚姻情况、教育水平、经济水平、地区、户籍、精神疾病家族史、历史暴力类型以及首次暴力的年龄,在结局发生时或最后一次随访记录收集或计算年龄、病程、未经治疗的时间、药物不良反应、住院情况、监禁情况。服药依从性和社会功能是本研究的动态变量,在每一次随访时收集。
1.3 统计学方法
使用R 4.4.2进行统计分析,使用的程序包括lcmm、pscl。在数据分析之前,首先使用基于随机森林的链式多重填补独立地对基线和随访记录中的静态变量缺失值进行填补,随后使用相关性矩阵判断研究变量间的共线性。所有检验均采用双侧检验,检验水准α=0.05。
1.3.1 动态模式识别
本研究首先采用生长混合模型识别研究对象的服药依从性与社会功能的变化模式。该模型是一种识别总体内多个异质性未观测类的非监督聚类分析方法[15],可用于描述每个未观测类随时间变化的模式,并检验未观测类之间的差异。本研究使用人时数计算的率来反映服药依从性和社会功能,在调整病情稳定性和病程后分别对其拟合不同类别数的随机斜率模型,选择调整后的贝叶斯信息准则(adjusted Bayesian information criterion, aBIC)较小、Lo-Mendell-Rubin调整似然比检验有统计学意义、熵>0.8的模型[16]。
1.3.2 预测模型建立
在识别服药依从性与社会功能的变化模式基础上,考虑到本研究数据的过离散与零膨胀现象,进一步选择零膨胀负二项回归模型建立预测模型[17],形成一种联合模型。为了比较该联合模型和传统静态模型的预测效能,两组预测模型使用了除服药依从性和社会功能外相同的协变量集,协变量集的提取是由联合模型通过逐步回归法确定的。静态模型中服药依从性和社会功能纳入结局发生时或最后一次随访记录,联合模型则使用生长混合模型识别的模式。零膨胀负二项回归模型的结果包括使用发生率比(incidence rate ratio, IRR)描述的计数模型和使用比值比(odds ratio, OR)描述的零膨胀模型,前者反映了暴力再犯的频率,后者反映了暴力再犯是否发生的可能性。
1.3.3 模型评价
模型拟合与预测采用10折训练-测试分割交叉验证框架,将数据按照7∶3随机划分为训练集与测试集进行内部验证,该方法保证了训练集与测试集具有良好的代表性。模型区分度通过绘制实际-预测值图评价,错误计数或分类的数量越少说明模型区分度越好;模型校准度采用赤池信息准则(Akaike information criterion, AIC)、均方根误差(root mean squared error, RMSE)以及平均绝对误差(mean absolute error, MAE)评价,数值越小说明模型校准度越好[18]。
2. 结果
共有157位(3.44%)研究对象在2017年1月–2018年6月之间发生了暴力再犯。与未发生暴力再犯的研究对象相比,发生暴力再犯的研究对象中平均年龄更小,男性构成比更高,未受教育构成比更高,药物不良反应构成比更高,住院构成比更低,其他研究变量差异无统计学意义(表1)。
表 14565 名社区精分患者暴力再犯组与非暴力再犯组一般资料比较Table 1. Comparison of general data between the recidivism group and the non-recidivism group of4565 SSDPVariable Recidivism
(n=157)Non-recidivism
(n=4408 )χ2/t P Age/yr., $ \bar {x}\pm s $ 48.92±13.67 52.51±14.09 2.645 0.008 Period/years, $ \bar {x}\pm s $ 17.53±11.99 18.14±11.56 0.649 0.516 Sex/case (%) 4.056 0.044 Male 85 (54.14) 2027 (45.98)Female 72 (45.86) 2381 (54.02)Marital status/case (%) 1.976 0.577 Single 46 (29.31) 1350 (30.63)Married 100 (63.69) 2616 (59.35)Diverse 5 (3.18) 192 (4.36) Widowed 6 (3.82) 250 (5.67) Education/case (%) 4.696 0.03 Uneducated 55 (35.05) 1198 (27.18)Educated 102 (64.95) 3210 (72.82)Economy/case (%) 0.114 0.735 Poverty 43 (27.39) 1262 (28.63)Non-poverty 114 (72.61) 3146 (71.37)Employment/case (%) 0.732 0.392 Unemployed 17 (10.83) 390 (8.85) Employed 140 (89.17) 4018 (91.15)Family history/case (%) 0.602 0.438 Yes 10 (6.37) 220 (4.99) No 147 (93.63) 4188 (95.01)Adverse drug reaction/case (%) 8.547a 0.003 Yes 7 (4.46) 58 (1.32) No 150 (95.54) 4350 (98.68)Crime history/case (%) 3.303a 0.192 VTO 8 (5.1) 251 (5.69) SH 0 87 (1.97) VTO+SH 149 (94.9) 4070 (92.34)Hospital/case (%) 4.155 0.042 Yes 4 (2.55) 292 (6.62) No 153 (97.45) 4116 (93.38)DUP/case (%) 2.231 0.135 <1 year 137 (87.26) 4002 (90.79)≥1 year 20 (12.74) 406 (9.21) Social functioning/case (%) 2.894 0.235 Good 26 (16.56) 774 (17.56) Medium 109 (69.43) 3199 (72.57)Bad 22 (14.01) 435 (9.87) Compliance/case (%) 4.968 0.174 Untreated 20 (12.74) 383 (8.69) Compliant 86 (54.78) 2702 (61.3)Intermittent 42 (25.75) 1022 (23.19)Non-compliant 9 (5.73) 301 (6.82) VTO: violence to other; SH: self harm; DUP: duration of untreated psychosis. a Adjusted χ2 using correction for continuity. 2.1 模式识别
比较模型参数后(表2),结合临床意义,服药依从性的4种模式(图1)分别为:①
1736 例研究对象(41.71%)始终维持在80%以下,表现为间断性服药,记为“持续不依从(persistently non-compliant, PNC)”;②252例研究对象(6.05%)前期较好,随时间逐渐下降至较低水平,表现为依从性恶化,记为“下降”;③1777 例研究对象(42.70%)始终维持在80%以上,记为“持续依从(persistently compliant, PC)”;④397例研究对象(9.54%)前期较差,随时间逐渐上升至较高水平,表现为依从性好转,记为“上升”。表 2 不同类别数的服药依从性随机斜率生长混合模型参数Table 2. Parameters of GMM for medication adherence of different categoriesClass number aBIC LRT Entropy Smallest sample size 1 − 33761.6 − − − 2 − 31864.2 P=0.009 0.76 1786 3 − 29755.8 P=0.006 0.82 664 4 − 27606.5 P=0.005 0.84 664 5 − 25855.1 P=0.009 0.84 252 6 − 26462.7 P=0.074 0.82 252 aBIC: adjusted Bayesian information criterion; LRT: Lo-Mendell-Rubin adjusted likelihood ratio test. 比较模型参数后(表3),社会功能的4种模式(图2):①962例研究对象(21.07%)稳定在较高水平,记为“持续较好(constantly stable, CS)”;②
3144 例研究对象(68.87%)稳定在中等水平,记为“持续中等(constantly medium, CM)”;③212例研究对象(4.64%)前期稳定在中等水平,后期稳定在较高水平,记为“上升”;④247例研究对象(5.17%)前期稳定在中等水平,后期稳定在较低水平,记为“下降”。表 3 不同类别数的社会功能随机斜率生长混合模型参数Table 3. Parameters of GMM for social functions of different categoriesClass number aBIC LRT Entropy Smallest sample size 1 − 39788.8 − − − 2 − 35524.2 P=0.001 0.79 1349 3 − 34029.1 P=0.004 0.79 1064 4 − 33002.6 P=0.004 0.81 212 5 − 32496.4 P=0.052 0.80 178 6 − 32302.2 P=0.107 0.81 178 aBIC: adjusted Bayesian information criterion; LRT: Lo-Mendell-Rubin adjusted likelihood ratio test. 2.2 预测模型
静态模型结果显示(表4):计数模型中,与不服药对照组相比,间断用药减少了暴力再犯的次数〔IRR=0.312,95%置信区间(confidence interval, CI):0.137,0.708〕;既往暴力类型为对他人暴力合并自身暴力相比仅有对他人暴力减少了暴力再犯的次数(IRR=0.113,95%CI:0.023,0.565)。零膨胀模型中,历史暴力类型、药物不良反应、社会功能和服药依从性是暴力再犯是否发生的预测因素。
表 44565 例社区精分患者静态模型与联合模型中计数模型以及零膨胀模型结果Table 4. IRR with 95% confidence interval in counting model and odds ratio with 95% confidence interval in zero-inflated model of4565 community SSDP in the baseline model and the joint modelVariable Factor Counting model Zero-inflated model Baseline model Joint model Baseline model Joint model Age 0.981 (0.953-1.010) 0.964 (0.938-0.990)* 1.009 (0.989-1.029) 0.976 (0.957-0.996)* Marital status Single Reference Reference Reference Reference Married 0.246 (0.038-1.600) 0.375 (0.208-0.678)* 1.696 (0.445-6.467) 0.813 (0.466-1.419) Divorce 0.687 (0.329-1.432) 3.77 (0.827-17.185) 1.108 (0.625-1.965) 2.408 (0.719-8.071) Widowed 1.026 (0.502-2.099) 4.031 (1.084-14.987)* 1.647 (0.915-2.965) 2.492 (0.915-6.784) Education Uneducated Reference Reference Reference Reference Educated 0.736 (0.131-4.124) 0.486 (0.267-0.885)* 0.405 (0.102-1.609) 0.786 (0.495-1.248) Economy Non-poverty Reference Reference Reference Reference Poverty 1.77 (0.943-3.321) 2.547 (1.042-6.226)* 1.138 (0.669-1.935) 0.664 (0.315-1.399) Crime history type VTO Reference Reference Reference Reference SH NAa NAa NAa NAa VTO+SH 0.113 (0.023-0.565)* 0.056 (0.013-0.235)* 0.221 (0.040-1.231) 0.163 (0.030-0.886)* Adverse drug reaction No Reference Reference Reference Reference Yes 1.025 (0.359-2.932) 1.891 (0.714-5.008) 8.525 (2.574-28.235)* 3.888 (1.482-10.199)* Compliance Untreated Reference Reference Compliant 0.563 (0.292-1.085) 2.275 (1.229-4.21)* Intermittent 0.312 (0.137-0.708)* 1.254 (0.664-2.366) Non-compliant 0.61 (0.321-1.158) 1.218 (0.694-2.137) Compliance pattern Untreated Reference Reference PNC 0.466 (0.225-0.966)* 1.855 (0.996-3.433) Descending 0.651 (0.174-2.433) 3.380 (1.157-9.876)* PC 0.315 (0.109-0.914)* 0.856 (0.463-1.580) Ascending 0.277 (0.124-0.618)* 1.645 (0.881-3.075) Social functioning Good Reference Reference Medium 0.559 (0.257-1.216) 0.481 (0.281-0.824)* Bad 0.175 (0.030-1.032) 0.076 (0.040-0.143)* Social functioning pattern CS Reference Reference CM 0.655 (0.355-1.207) 1.394 (0.851-2.284) Ascending 0.744 (0.394-1.404) 0.550 (0.173-1.753) Descending 2.901 (0.770-10.934) 5.259 (2.547-10.861)* VTO: violence to other; SH: self harm; PNC: persistently non-compliant; PC: persistently compliant; CS: constantly stable; CM: constantly medium. a The count contains zero. * P<0.05. 联合模型结果显示(表4):计数模型中,随着年龄的增加暴力再犯的次数减少(IRR=0.964,95%CI:0.938,0.99);与未婚对照组相比,已婚(IRR=0.375,95%CI:0.208,0.678)暴力再犯的次数减少,而丧偶(IRR=4.031,95%CI:1.084,14.987)暴力再犯的次数增加;受教育有利于减少暴力再犯次数(IRR=0.486,95%CI:0.267,0.885);贫困会增加暴力再犯次数(IRR=2.547,95%CI:1.042,6.226);与不服药对照组相比,持续不依从(IRR=0.466,95%CI:0.225,0.966)、持续依从(IRR=0.315,95%CI:0.109,0.914)和依从性上升(IRR=0.277,95%CI:0.124,0.618)均有利于减少暴力再犯次数;历史暴力类型为对他人暴力合并自身暴力相比仅有对他人暴力减少了暴力再犯的次数(IRR=0.056,95%CI:0.013,0.235)。零膨胀模型中,年龄、历史暴力类型、药物不良反应、服药依从性模式和社会功能模式是暴力再犯是否发生的预测因素。
表 5 静态模型与联合模型在训练集、测试集中的拟合或预测效果评价指标Table 5. The evaluation indicators for the fitting or prediction performance of static model and joint model in the train and test setsModel Set AIC RMSE MAE Baseline model Train 963.2±32.6 0.313±0.033 0.266±0.031 Test 0.292±0.028 0.204±0.021 Joint model Train 776.5±26.4 0.171±0.014 0.131±0.018 Test 0.168±0.013 0.131±0.018 AIC: Akaike information criterion; RMSE: root mean squared error; MAE: mean absolute error. 2.3 模型验证
实际-预测值图(图3)显示联合模型在不同暴力再犯计数下的错误计数或分类均更少。交叉验证结果(表5)显示静态模型训练集AIC平均值为963.2±13.6,联合模型训练集AIC平均值为776.5±9.4;静态模型测试集RMSE平均值为0.292±0.028,MAE平均值0.204±0.021,联合模型测试集RMSE平均值为0.168±0.013,MAE平均值0.131±0.018。
3. 讨论
本研究充分关注公共卫生资源相对匮乏的基层公共卫生组织管理下的社区精分患者,创新性地使用了生长混合模型和零膨胀负二项回归模型联合建模的方法,一方面识别动态变量变化模式,为开展个体化综合性康复措施提供直接证据;另一方面识别暴力再犯相关预测因素,为动态变量的识别与处理提供全新统计分析策略,并填补国内相关领域实证研究空白,进一步为推动基层公共卫生综合干预体系建设提供理论依据。
本研究结果显示,与静态模型相比,联合模型训练集的AIC平均值以及测试集的RMSE和MAE平均值都更小,95%CI未出现重合,提示联合模型预测性能更好[18],因此可以认为联合建模是一种有效的动态变量识别与处理统计学方法。此外,无论是计数模型还是零膨胀模型,联合模型都比静态模型识别出了更多的预测变量,并且静态模型中服药依从性和社会功能与暴力再犯的关系与既往研究存在明显差异[4, 12],提示使用静态模型识别和处理动态变量可能会得出错误的统计学结论。同时,联合建模避免了因排除缺失值而损失样本量或因多重插补而改变统计学效应的局限性。
服药依从性与社会功能作为基层公共卫生治疗与康复的工作重点,研究不同个体的发展模式具有重要意义。本研究使用了基于人时数计算的率来识别服药依从性和社会功能的模式,将随访时间间隔内离散的分类变量转化为了更具有实际意义的连续型变量,调整病情稳定性和病程后服药依从性与社会功能被识别出类似的4种模式。值得关注的是,社区患者中有相当比例的个体在研究期间出现服药依从性和社会功能的快速恶化,研究末期甚至低于持续不依从或社会功能持续中等的水平,这可能与现有药物使用模式无反应有关[19],因此应当对这部分患者采取对应的社区综合性康复措施以恢复其服药依从性和社会功能。
与既往研究结论相同,本研究使用联合模型得到的暴力再犯预测因素与现有关于住院患者或司法监禁患者存在较大差异。计数模型中,与未婚相比,已婚降低暴力再犯次数而离异增加暴力再犯次数,这可能与婚姻提供了一定的社会支持有关[20],而离异作为一种生活负性经历独立影响暴力再犯;受教育的个体往往具备基本的社会常识与法律常识,对自身行为的后果有一定认知能力,因此受教育可以降低暴力再犯次数;贫困影响了社区患者的社会支持以及卫生服务的可及性[21],因此会增加暴力再犯的次数。对于服药依从性,依从性下降与暴力再犯的次数无关,这同样可能与治疗无反应有关[19],而依从性上升、持续依从和持续不依从均能减少暴力再犯的次数,提示及时开展抗精神病药物治疗的重要作用。既往同时存在自我暴力和对他人暴力的患者被司法系统重点关注,暴力再犯的成本降低,同时这部分患者可能存在一种情绪性限制,这些限制帮助减少暴力再犯次数。零膨胀模型中,年龄、既往暴力类型与计数模型的结论基本一致。值得注意的是,服药依从性下降是暴力再犯的独立危险因素。同时,具有药物不良反应被认为是暴力再犯的危险因素,因为不良反应增加了药物使用的负担。而对于社会功能,社会功能下降被认为是暴力再犯的危险因素,社会功能的快速恶化可能与认知功能下降相关[22],也可能与不充分的康复措施有关。因此,与生长混合模型的结论一致,对于服药依从性和社会功能快速恶化的个体,社区应该给予更多关注。
本研究的局限性包括:①需要进一步使用来自不同地区的数据库进行外部验证以保证推广性;②受数据库指标限制,未纳入其他可能与暴力再犯相关的研究变量,例如认知功能等,可能导致研究结论有限;③对于出现多次暴力再犯的个体,其首次暴力再犯后的服药依从性和社会功能被识别为缺失值。尽管在本研究中这部分个体基本没有被错误分类,但是缺失值可能对生长混合模型总体分类能力造成影响。
* * *
作者贡献声明 吴祥瑞负责论文构思、数据审编、正式分析、研究方法和初稿写作,杨先梅负责提供资源、监督指导和审读与编辑写作,范箬馨负责提供资源和监督指导,刘军和向虎负责研究项目管理,左传隆和刘祥负责软件,刘元元负责审读与编辑写作。所有作者已经同意将文章提交给本刊,且对将要发表的版本进行最终定稿,并同意对工作的所有方面负责。
Author Contribution WU Xiangrui is responsible for conceptualization, data curation, formal analysis, methodology, and writing--original draft. YANG Xianmei is responsible for resources, supervision, and writing--review and editing. FAN Ruoxin is responsible for resources and supervision. LIU Jun and XIANG Hu are responsible for project administration. ZUO Chuanlong and LIU Xiang are responsible for software. LIU Yuanyuan is responsible for writing--review and editing. All authors consented to the submission of the article to the Journal. All authors approved the final version to be published and agreed to take responsibility for all aspects of the work.
利益冲突 所有作者均声明不存在利益冲突
Declaration of Conflicting Interests All authors declare no competing interests.
-
表 1
4565 名社区精分患者暴力再犯组与非暴力再犯组一般资料比较Table 1 Comparison of general data between the recidivism group and the non-recidivism group of
4565 SSDPVariable Recidivism
(n=157)Non-recidivism
(n=4408 )χ2/t P Age/yr., $ \bar {x}\pm s $ 48.92±13.67 52.51±14.09 2.645 0.008 Period/years, $ \bar {x}\pm s $ 17.53±11.99 18.14±11.56 0.649 0.516 Sex/case (%) 4.056 0.044 Male 85 (54.14) 2027 (45.98)Female 72 (45.86) 2381 (54.02)Marital status/case (%) 1.976 0.577 Single 46 (29.31) 1350 (30.63)Married 100 (63.69) 2616 (59.35)Diverse 5 (3.18) 192 (4.36) Widowed 6 (3.82) 250 (5.67) Education/case (%) 4.696 0.03 Uneducated 55 (35.05) 1198 (27.18)Educated 102 (64.95) 3210 (72.82)Economy/case (%) 0.114 0.735 Poverty 43 (27.39) 1262 (28.63)Non-poverty 114 (72.61) 3146 (71.37)Employment/case (%) 0.732 0.392 Unemployed 17 (10.83) 390 (8.85) Employed 140 (89.17) 4018 (91.15)Family history/case (%) 0.602 0.438 Yes 10 (6.37) 220 (4.99) No 147 (93.63) 4188 (95.01)Adverse drug reaction/case (%) 8.547a 0.003 Yes 7 (4.46) 58 (1.32) No 150 (95.54) 4350 (98.68)Crime history/case (%) 3.303a 0.192 VTO 8 (5.1) 251 (5.69) SH 0 87 (1.97) VTO+SH 149 (94.9) 4070 (92.34)Hospital/case (%) 4.155 0.042 Yes 4 (2.55) 292 (6.62) No 153 (97.45) 4116 (93.38)DUP/case (%) 2.231 0.135 <1 year 137 (87.26) 4002 (90.79)≥1 year 20 (12.74) 406 (9.21) Social functioning/case (%) 2.894 0.235 Good 26 (16.56) 774 (17.56) Medium 109 (69.43) 3199 (72.57)Bad 22 (14.01) 435 (9.87) Compliance/case (%) 4.968 0.174 Untreated 20 (12.74) 383 (8.69) Compliant 86 (54.78) 2702 (61.3)Intermittent 42 (25.75) 1022 (23.19)Non-compliant 9 (5.73) 301 (6.82) VTO: violence to other; SH: self harm; DUP: duration of untreated psychosis. a Adjusted χ2 using correction for continuity. 表 2 不同类别数的服药依从性随机斜率生长混合模型参数
Table 2 Parameters of GMM for medication adherence of different categories
Class number aBIC LRT Entropy Smallest sample size 1 − 33761.6 − − − 2 − 31864.2 P=0.009 0.76 1786 3 − 29755.8 P=0.006 0.82 664 4 − 27606.5 P=0.005 0.84 664 5 − 25855.1 P=0.009 0.84 252 6 − 26462.7 P=0.074 0.82 252 aBIC: adjusted Bayesian information criterion; LRT: Lo-Mendell-Rubin adjusted likelihood ratio test. 表 3 不同类别数的社会功能随机斜率生长混合模型参数
Table 3 Parameters of GMM for social functions of different categories
Class number aBIC LRT Entropy Smallest sample size 1 − 39788.8 − − − 2 − 35524.2 P=0.001 0.79 1349 3 − 34029.1 P=0.004 0.79 1064 4 − 33002.6 P=0.004 0.81 212 5 − 32496.4 P=0.052 0.80 178 6 − 32302.2 P=0.107 0.81 178 aBIC: adjusted Bayesian information criterion; LRT: Lo-Mendell-Rubin adjusted likelihood ratio test. 表 4
4565 例社区精分患者静态模型与联合模型中计数模型以及零膨胀模型结果Table 4 IRR with 95% confidence interval in counting model and odds ratio with 95% confidence interval in zero-inflated model of
4565 community SSDP in the baseline model and the joint modelVariable Factor Counting model Zero-inflated model Baseline model Joint model Baseline model Joint model Age 0.981 (0.953-1.010) 0.964 (0.938-0.990)* 1.009 (0.989-1.029) 0.976 (0.957-0.996)* Marital status Single Reference Reference Reference Reference Married 0.246 (0.038-1.600) 0.375 (0.208-0.678)* 1.696 (0.445-6.467) 0.813 (0.466-1.419) Divorce 0.687 (0.329-1.432) 3.77 (0.827-17.185) 1.108 (0.625-1.965) 2.408 (0.719-8.071) Widowed 1.026 (0.502-2.099) 4.031 (1.084-14.987)* 1.647 (0.915-2.965) 2.492 (0.915-6.784) Education Uneducated Reference Reference Reference Reference Educated 0.736 (0.131-4.124) 0.486 (0.267-0.885)* 0.405 (0.102-1.609) 0.786 (0.495-1.248) Economy Non-poverty Reference Reference Reference Reference Poverty 1.77 (0.943-3.321) 2.547 (1.042-6.226)* 1.138 (0.669-1.935) 0.664 (0.315-1.399) Crime history type VTO Reference Reference Reference Reference SH NAa NAa NAa NAa VTO+SH 0.113 (0.023-0.565)* 0.056 (0.013-0.235)* 0.221 (0.040-1.231) 0.163 (0.030-0.886)* Adverse drug reaction No Reference Reference Reference Reference Yes 1.025 (0.359-2.932) 1.891 (0.714-5.008) 8.525 (2.574-28.235)* 3.888 (1.482-10.199)* Compliance Untreated Reference Reference Compliant 0.563 (0.292-1.085) 2.275 (1.229-4.21)* Intermittent 0.312 (0.137-0.708)* 1.254 (0.664-2.366) Non-compliant 0.61 (0.321-1.158) 1.218 (0.694-2.137) Compliance pattern Untreated Reference Reference PNC 0.466 (0.225-0.966)* 1.855 (0.996-3.433) Descending 0.651 (0.174-2.433) 3.380 (1.157-9.876)* PC 0.315 (0.109-0.914)* 0.856 (0.463-1.580) Ascending 0.277 (0.124-0.618)* 1.645 (0.881-3.075) Social functioning Good Reference Reference Medium 0.559 (0.257-1.216) 0.481 (0.281-0.824)* Bad 0.175 (0.030-1.032) 0.076 (0.040-0.143)* Social functioning pattern CS Reference Reference CM 0.655 (0.355-1.207) 1.394 (0.851-2.284) Ascending 0.744 (0.394-1.404) 0.550 (0.173-1.753) Descending 2.901 (0.770-10.934) 5.259 (2.547-10.861)* VTO: violence to other; SH: self harm; PNC: persistently non-compliant; PC: persistently compliant; CS: constantly stable; CM: constantly medium. a The count contains zero. * P<0.05. 表 5 静态模型与联合模型在训练集、测试集中的拟合或预测效果评价指标
Table 5 The evaluation indicators for the fitting or prediction performance of static model and joint model in the train and test sets
Model Set AIC RMSE MAE Baseline model Train 963.2±32.6 0.313±0.033 0.266±0.031 Test 0.292±0.028 0.204±0.021 Joint model Train 776.5±26.4 0.171±0.014 0.131±0.018 Test 0.168±0.013 0.131±0.018 AIC: Akaike information criterion; RMSE: root mean squared error; MAE: mean absolute error. -
[1] WHITING D, LICHTENSTEIN P, FAZEL S. Violence and mental disorders: a structured review of associations by individual diagnoses, risk factors, and risk assessment. Lancet Psychiatry, 2021, 8(2): 150–161. doi: 10.1016/S2215-0366(20)30262-5.
[2] WHITING D, GULATI G, GEDDES J R, et al. Association of schizophrenia spectrum disorders and violence perpetration in adults and adolescents from 15 countries a systematic review and meta-analysis. JAMA Psychiatry, 2022, 79(2): 120–132. doi: 10.1001/jamapsychiatry.2021.3721.
[3] HE Y, GU Y, YU M L, et al. Research on interpersonal violence in schizophrenia: based on different victim types. BMC Psychiatry, 2022, 22(1): 172. doi: 10.1186/s12888-022-03820-7.
[4] BO S N, ABU-AKEL A, KONGERSLEV M, et al. Predictors of criminal offending in a clinical sample of patients diagnosed with schizophrenia: a 6-year follow-up study. Personal Disord, 2021, 12(3): 216–227. doi: 10.1037/per0000401.
[5] LIN I, MUSER E, MUNSELL M, et al. Economic impact of psychiatric relapse and recidivism among adults with schizophrenia recently released from incarceration: a Markov model analysis. J Med Echo, 2015, 18(3): 219–229. doi: 10.3111/13696998.2014.971161.
[6] 刘建梅, 吕盼, 胡峻梅. 156例重复违法的精神障碍患者特征分析. 法医学杂志, 2013, 29(4): 278–281. doi: 10.3969/j.issn.1004-5619.2013.04.011. LIU J M, LYU P, HU J M. Characteristics of recidivism in patients with mental disorders: 156 cases analysis. J Forensic Med, 2013, 29(4): 278–281. doi: 10.3969/j.issn.1004-5619.2013.04.011.
[7] ADILY A, ALBALAWI O, KARIMINIA A, et al. Association between early contact with mental health services after an offense and reoffending in individuals diagnosed with psychosis. JAMA Psychiatry, 2020, 77(11): 1137–1146. doi: 10.1001/jamapsychiatry.2020.1255.
[8] LAMBERTI J S, KATSETOS V, JACOBOWITZ D B, et al. Psychosis, mania and criminal recidivism: associations and implications for prevention. Harv Rev Psychiatry, 2020, 28(3): 179–202. doi: 10.1097/HRP.0000000000000251.
[9] THOMSON L, REES C. Long-term outcomes of the recovery approach in a high-security mental health setting: a 20 year follow-up study. Front Psychiatry, 2023, 14: 1111377. doi: 10.3389/fpsyt.2023.1111377.
[10] ONG K, CARROLL A, REID S, et al. Community outcomes of mentally disordered homicide offenders in Victoria. Aust N Z Psychiatry, 2009, 43(8): 775–780. doi: 10.1080/00048670903001976.
[11] TABITA B, De SANTI M G, KJELLIN L. Criminal recidivism and mortality among patients discharged from a forensic medium secure hospital. Nord J Psychiatry, 2012, 66(4): 283–289. doi: 10.3109/08039488.2011.644578.
[12] OUESLATI B, FEKIH-ROMDHANE F, MRABET A, et al. Correlates of offense recidivism in patients with schizophrenia. Int J Law Psychiatry, 2018, 58: 178–183. doi: 10.1016/j.ijlp.2018.05.001.
[13] MICHEL S F, RIAZ M, WEBSTER C, et al. Using the HCR-20 to predict aggressive behavior among men with schizophrenia living in the community: accuracy of prediction, general and forensic settings, and dynamic risk factors. Int J Forensic Ment Psychiatry, 2013, 12(1): 1–13. doi: 10.1080/14999013.2012.760182.
[14] DACK C, ROSS J, PAPADOPOULOS C, et al. A review and meta-analysis of the patient factors associated with psychiatric in-patient aggression. Acta Psychiatr Scand, 2013, 127(4): 255–268. doi: 10.1111/acps.12053.
[15] Van der NEST G, PASSOS V L, CANDEL M, et al. An overview of mixture modelling for latent evolutions in longitudinal data: Modelling approaches, fit statistics and software. Adv Life Course Res, 2020, 43: 100323. doi: 10.1016/j.alcr.2019.100323.
[16] MARCOULIDES K M, TRICHERA L. Detecting unobserved heterogeneity in latent growth curve models. Struct Equ Modeling, 2019, 26(3): 390–401. doi: 10.1080/10705511.2018.1534591.
[17] LORD D, WASHINGTON S P, IVAN J N. Poisson, Poisson-gamma and zero-inflated regression models of motor vehicle crashes: balancing statistical fit and theory. Accdi Anal Prev, 2005, 37(1): 35–46. doi: 10.1016/j.aap.2004.02.004.
[18] CHAI T, DRAXLER R R. Root mean square error (RMSE) or mean absolute error (MAE)? - Arguments against avoiding RMSE in the literature. Geosci Model Dev, 2014, 7(3): 1247–1250. doi: 10.5194/gmd-7-1247-2014.
[19] LONG Y X, TONG X L, AWAD M, et al. Violence, runaway, and suicide attempts among people living with schizophrenia in China: Prevalence and correlates. PeerJ, 2022, 10: e13033. doi: 10.7717/peerj.13033.
[20] PESCOSOLIDO B A, HALPERN-MANNERS A, LUO L Y, et al. Trends in public stigma of mental illness in the US, 1996-2018. JAMA Netw Open, 2021, 4(12): e2140202. doi: 10.1001/jamanetworkopen.2021.40202.
[21] TSIGEBRHAN R, SHIBRE T, MEDHIN G, et al. Violence and violent victimization in people with severe mental illness in a rural low-income country setting: a comparative cross-sectional community study. Schizophr Res, 2014, 152(1): 275–282. doi: 10.1016/j.schres.2013.10.032.
[22] IOZZINO L, HARVEY P D, CANESSA N, et al. Neurocognition and social cognition in patients with schizophrenia spectrum disorders with and without a history of violence: results of a multinational European study. Ttansl Psychiatry, 2021, 11(1): 620. doi: 10.1038/s41398-021-01749-1.
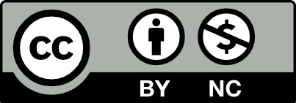
开放获取 本文遵循知识共享署名—非商业性使用4.0国际许可协议(CC BY-NC 4.0),允许第三方对本刊发表的论文自由共享(即在任何媒介以任何形式复制、发行原文)、演绎(即修改、转换或以原文为基础进行创作),必须给出适当的署名,提供指向本文许可协议的链接,同时标明是否对原文作了修改;不得将本文用于商业目的。CC BY-NC 4.0许可协议详情请访问 https://creativecommons.org/licenses/by-nc/4.0